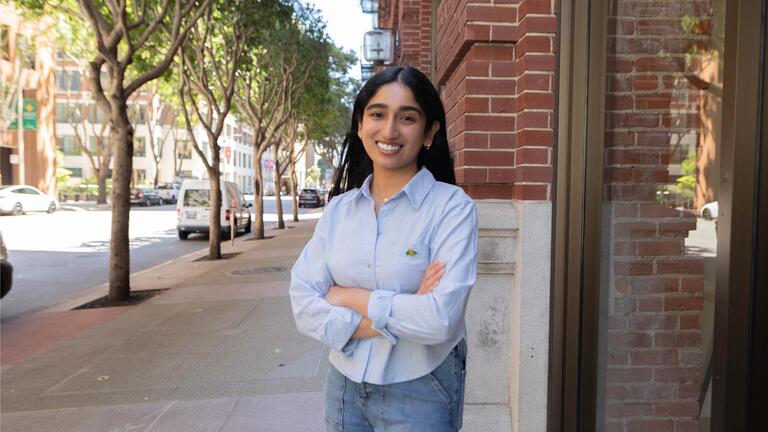
Practicum
All students gain real-world experience for approximately nine months of the program (16 hours/week) tackling data science and analytics problems at organizations around the San Francisco Bay Area and beyond. Each year, roughly 50 companies pitch their projects to our students, and students rank their preferences based on the project proposals (past and current partners include those shown below). Students with complementary strengths are matched up to form a team. To ensure the success of the projects, each team is actively mentored by a faculty member and a company mentor who participate in regular meetings to supervise and provide technical and mathematical expertise.
Hands-on Experience with Industry Leaders
Following an initial hypothesis, students typically engage in data acquisition, exploratory data analysis, feature extraction, model development and evaluation, as well as oral and written communication of results. Class schedules are set so that students work on their projects two dedicated days per week throughout the practicum.
- Practicum begins in mid-October.
- Students devote 16 hours a week to practicum work on average.
- Projects may be paid or unpaid.
How Can Your Organization Participate?
Interested in working with a group of highly motivated and committed students from this exciting graduate program?
Partnerships
-
A select list:
- American Civil Liberties Union (ACLU) Foundation of Northern California
- Bay Area Rapid Transit (BART)
- BlackRock
- Blueboard
- Boost Sport
- California Forward
- Cerenetics
- Environmental Defense Fund
- California Department of Fisheries and Wildlife
- First Republic Bank
- Freedom Financial Network
- Golden State Warriors
- Hims & Hers
- Metaphor Data
- Metromile
- Nextracker
- Nisum
- New York Mets
- Oportun
- Orange Silicon Valley
- Pocket Gems
- Propeller Health
- Recology
- Reputation
- Stanford Graduate School of Business
- Stanford Medicine
- SubWiFi
- Target
- The Nature Conservancy
- UCSF Department of Radiation Oncology
- UCSF Brain Networks Laboratory
- UCSF Bakar Computational Health Sciences Institute (Gastro)
- UCSF Hospital Medicine
- Velux
- W.L. Gore & Associates
Past Projects
-
American Civil Liberties Union of Northern California (ACLU)
Our Team: Ian Duke, Felix Tong
Faculty Mentor(s): Robert Clements
Company Liaison(s): Dylan Verner-Crist, John Do, Emi YoungStudents spearheaded the development and implementation of scalable models in Python using advanced natural language processing and machine learning techniques to automate the analysis of unstructured video data from over 500 police traffic stops. They built a data pipeline that integrates open-source machine learning models, transcription techniques, and data storage methods to associate 3,500 written reports with corresponding body camera videos, efficiently organizing the stored data. The team led an initiative to conduct time series forecasting analyses and observational studies exploring the impact of facial recognition technology on crime and clearance rates in jurisdictions across California. Additionally, they regularly created data visualizations for the office’s Lead Investigator and explained findings and analytical approaches to non-technical legal professionals.
AERO
Our Team: Colin Bennie, Yen-Hsin Fang
Faculty Mentor(s): Victor Palacios
Company Liaison(s): Ray YockeStudents led the design and implementation of machine-learning-focused Python modules, leveraging XGBoost and cron automations to improve prediction accuracy for sales performance across the route network, as well as increasing revenues through precise demand predictions. The team collaborated closely with executives to gain insights into organizational concerns and designed machine learning solutions to address data-level challenges, establishing data-driven decision-making processes throughout the organization. They created and maintained a pipeline to integrate over 1 million third-party records into existing data used in machine learning approaches, significantly enhancing model performance.
American School of Dubai
Our Team: Brandon Hom
Faculty Mentor(s): Mahesh Chaudhari
Company Liaison(s): Ken SimondsUtilizing BigQuery and Looker Studio, the team analyzed and summarized over 60,000 data points related to academic performance and reading habits, yielding valuable insights for school leadership. They streamlined data scraping pipelines in Airflow, significantly reducing code complexity by consolidating more than 30 pipeline files into a single file and expediting the implementation of new pipelines. Additionally, they designed an Airflow data pipeline to extract raw usage logs from Looker Studio, resulting in the development of a dashboard that provides insights on dashboard usage. This innovation led to an 85% reduction in costs and saved over 50 hours annually.
Atlassian
Our Team: Jiaxuan Ouyang, Sissi Shen, Laila Zaidi
Faculty Mentor(s): Robert Clements
Company Liaison(s): Steve ShibuyaThe team developed a validation package, achieving a 90% reduction in validation time and streamlining the process by reducing the codebase. They engineered and deployed a validation data pipeline in Databricks Cloud, which streamlined the assessment of models by utilizing PySpark and SQL to query large datasets containing over 20 million rows of marketing data. Leading the agile development process, the team managed project timelines using JIRA software. In their MLOps model governance efforts, they conducted performance tracking, drift detection, and time series analysis for different model versions, while organizing and managing these versions on MLFlow by logging metrics and tagging models according to their production status. Additionally, they collaborated with the data engineering team to derive new model features, resulting in a 5% increase in model performance.
Boston Children’s Hospital
Our Team: Amadeo Cabanela, Nathan Holmes-King, Sonal Shad
Faculty Mentor(s): William Bosl
Company Liaison(s): William Bosl, Andrew KissThe team developed feature extraction methods, including tensor factorization, to identify latent features associated with Rolandic Epilepsy in children. They employed machine learning techniques for seizure forecasting by analyzing brain activity data. This approach aimed to estimate the probability of a Rolandic seizure occurring, offering a more nuanced prediction than a simple binary outcome.
Buck Institute
Our Team: Samuel Campione, Zhiyuan (Freeman) Chen
Faculty Mentor(s): Daniel O'Connor
Company Liaison(s): Kai ZhouThe team developed single and double U-Net Convolutional Neural Network (CNN) models for identifying mitochondria in Drosophila brain scans, achieving a 94% accuracy rate. They implemented parallel computing and an image segmentation pipeline across various projects, including a 60% reduction in runtime for a cell segmentation pipeline using Joblib, leading to notable improvements in performance and reliability. Additionally, they conducted a comprehensive analysis tracking 21 ribosome paralogs across species to compare their conservation and evolutionary adaptations, assessing genetic diversity and age-related changes.
California Academy of Sciences
Our Team: Maricela Abarca, Aryan Mistry
Faculty Mentor(s): Shan Wang
Company Liaison(s): Joe RussackThe team guided a $50,000 investment in computing infrastructure by analyzing over 28 million server usage records with PySpark and a custom optimization algorithm. They automated a reporting system using APIs and MySQL, leading to an 88% increase in recognition for staff and their scientific publications. Additionally, they promoted the adoption of best practice identifiers to enhance the visibility of research output.
California Association of Food Banks
Our Team: Tyler Kahn
Faculty Mentor(s): Diane Woodbridge
Company Liaison(s): May Lynn TanThe team developed a data pipeline to collect, pre-process, and analyze US national census data, and created food insecurity and demographic dashboards in Tableau, which are used by California food banks and the State Assembly. This effort reduced manual labor by 80%. They also designed and implemented the company’s data ingestion pipeline and data warehouse using Google Cloud Platform, including Cloud Storage, Functions, Scheduler, and BigQuery. Additionally, the team worked closely with the CFO and Director of Research to identify data sources for exploration and analysis, offering guidance on tools and strategies to effectively leverage the available data.
California Data Collaborative
Our Team: Daniel Gonzalez
Faculty Mentor(s): Stephen Devlin
Company Liaison(s): Christopher Tull, David Marulli, Dan WangThe team developed and fine-tuned a leak detection algorithm using Snowflake and Python to analyze hourly water meter readings across various California water districts. They successfully identified and categorized leak patterns, which helped in reducing wastewater. They also implemented advanced data pre- and post-processing techniques to clean and structure time series data, improving the accuracy of a machine learning model for predicting water usage anomalies. The team aims to integrate a predictive module into the leak detection system to assist districts with real-time monitoring and timely management of leaks and usage anomalies.
Data Knobs
Our Team: Yan Ho (Mark) Lam, Thanh Dung (Zoe) Le, Ranjeet Nagarkar
Faculty Mentor(s): Daniel O'Connor
Company Liaison(s): Prashant DhingraThe team built a data pipeline for text-to-image and image-to-image semantic search using GCP, Google Vision AI, CLIP Model, and Pinecone. They deployed a RESTful API with FastAPI, achieving a high precision with an average cosine similarity score of 0.72. They also enabled seamless unstructured data extraction from PDFs and other document formats, such as PPTX and DOCX, using Langchain, Unstructured.IO, and other open-source libraries.
Dynetics
Our Team: Sung Hyun (James) Chung, Nithin Kuma
Faculty Mentor(s): Victor Palacios
Company Liaison(s): Cannot discloseTop secret government project. Details cannot be shared.
Environmental Defense Fund
Our Team: Karthik Ayyalasomayajula
Faculty Mentor(s): Daniel Jerison
Company Liaison(s): Dr. Chris CusackThe team engineered robust data pipelines for processing video and image data, optimizing them for machine learning and computer vision applications. They developed sampling and annotation scripts to support machine learning model validations by human reviewers, enhancing the accuracy of computer vision algorithm assessments. Additionally, they utilized Monte Carlo simulations to predict and visualize potential boat traffic patterns, contributing to environmental impact analysis.
Eventbrite
Our Team: Irene Garcia Montoya, Cassandra Richter
Faculty Mentor(s): Victor Palacios
Company Liaison(s): Jared Lauber, Chelsea Begrowicz, Katie PickettStudents developed a dashboard to proactively flag users based on revenue risk, which eliminated approximately 400 hours of manual tasks annually and improved model accuracy. They also created a discrepancy detection data pipeline to identify and quantify the impact of cross-database discrepancies in financial data. In addition, they initiated an early churn detection model for key clients.
Federal Home Loan Bank of San Francisco
Our Team: Vineeth Gupta Bodla
Faculty Mentor(s): Stephen Devlin
Company Liaison(s): Jason Lee, Jason Mora, Xu LiuThe team engineered complex SQL queries, optimized data pipelines, and applied text mining and data mining techniques to monitor 150 member KPIs. They performed exploratory data analysis (EDA) and hypothesis testing to compare traditional credit scoring frameworks with the VantageScore framework, identifying the potential to expand the customer base by 33 million. They developed a climate risk framework, orchestrated ETL processes, and created Power BI dashboards for strategic decision-making on assets. Additionally, they developed an interactive large language model (LLM) based dashboard in production, integrating sentiment evaluation of articles and media agencies and mapping stock price movements to monitor the reputation of top bank members.
Give Us The Floor
Our Team: Ireri Lisset Avila
Faculty Mentor(s): Victor Palacios
Company Liaison(s): Dr. Tessa Capelle, Adrian Ulsted, Valerie Grison-AslopThe team designed and implemented machine learning models using NLP techniques and transformers for binary classification. This reduced human intervention by 90%, saving $90K per year and benefiting over 2,000 users. They improved message retrieval through API requests by 80% with a Python script for effective date filtering across 400K messages. Additionally, they created an Apache Airflow DAG for scheduled runs of the entire pipeline, from data fetching to final classification.
How We Feel
Our Team: Jaywook Chung, Rashmi Panse
Faculty Mentor(s): David Guy Brizan
Company Liaison(s): James Regan, David ChengThe team developed a churn prediction model and designed A/B testing to improve retention, generating actionable insights projected to save $100K in annual marketing spend. They built a recommender system using the PyTorch framework to enhance personalized user experience by recommending in-app tools, which is expected to increase user engagement by 30%. Additionally, they transformed the data infrastructure by implementing ETL pipelines from MixPanel to a Google Cloud database, managing and querying over 100,000 event records in BigQuery SQL, saving more than 100 hours annually.
ISAZI
Our Team: Teja Davuluri, Varsha Moturi
Faculty Mentor(s): Mustafa Hajij
Company Liaison(s): Tanaka Chiromo, Dr. Brian WigdorowitzStudents developed a transformer-based forecasting model with inventory optimization algorithms to determine optimal stock levels, reorder points, and inventory allocation across a retail network of 200 stores, achieving a 15% reduction in purchasing costs. They increased forecast accuracy by 20% by fine-tuning Lag-Llama on over five years of sales data, modifying the architecture and temporal modeling.
LexisNexis
Our Team: Inseong Han, Sai Vamsi Pujari
Faculty Mentor(s): Victor Palacios
Company Liaison(s): Felipe Ferreira, Michelle JanneyCoyle, Yuhan (Hanna) WangThe team engineered advanced distributed preprocessing in Scala to optimize the analysis of 3.5 million entities (approximately 17 GB), driving large-scale data insights and feature engineering. They independently developed a semantic search engine, enhancing search capabilities beyond lexical search for an improved user experience. Additionally, they engineered an LLM-based chat application using the RAG framework for efficient entity retrieval from an internal knowledge base. They established a comprehensive Data Version Control pipeline to meticulously track data and experiment artifacts from inception to deployment. The team also architected the project repository using registry and builder design patterns, streamlining the management of runnable chains with Langchain-core and Langgraph.
Metaphor Data
Our Team: Mohan Rishi, Ronel Solomon
Faculty Mentor(s): Victor Palacios
Company Liaison(s): Mars Lan, Kirit Basu, Seyi AniganThe team developed a Slack bot using Generative AI and Vector Search indexes, retrieving and summarizing over 1,000 conversations to identify institutional knowledge threads and integrating sentiment analysis for insights. They implemented a Slack API with keyword-matching algorithms, boosting thread engagement and user interaction, leading to a 15% increase in user satisfaction. Additionally, they created open-source Python connectors for document sources and a TypeScript ingestion backend, and built production document vector embedding pipelines using Azure OpenAI, large language models, and MongoDB vector search to enhance the retrieval-augmented generation (RAG) process.
Metropolitan Transportation Commission (MTC)
Our Team: Manas, Nambiar, Evan Turkon
Faculty Mentor(s): Shan Wang
Company Liaison(s): Kaya Tollas, Kearey Smith, Aksel OlsenThe team leveraged Large Language Model (LLM) embeddings and prompt engineering techniques to analyze and synthesize insights from hundreds of thousands of public comments, significantly reducing analysis time and saving thousands of hours of manual labor. They engineered a high-performance API on AWS Lambda for the Doorway Affordable Housing Portal, enabling precise applicant eligibility verification via a spatial join SQL query on AWS Redshift, and conducted comprehensive evaluations to ensure speed and efficiency. Additionally, they designed and implemented an ETL data pipeline using AWS Redshift for Lyft’s Bay Wheels operation, managing tens of millions of rows of trip and station data, and conducted in-depth data analysis with Tableau. They developed a Python-based automation system using OpenAI’s API Platform to streamline sentiment analysis, topic tagging, and subtopic extraction for Plan Bay Area 2050+, reducing processing time by 98%. The team optimized OpenAI model performance through prompt engineering and data manipulation, reducing the Miscellaneous classification rate by 60%. They also designed an AWS Lambda function for geospatial joins in Amazon Redshift, performing rigorous testing to determine efficient methods for extracting GIS application layers.
Numeraxial LLC
Our Team: Tri Cao, Obtain Zandian
Faculty Mentor(s): Mahesh Chaudhari
Company Liaison(s): Jean NdoutoumouThe team engineered a Deep Ensemble Reinforcement Learning architecture integrating A2C, DDPG, PPO, and TD3 agents for the autonomous optimization of financial actions, resulting in a greater than 10% increase in ROI through adaptive policy learning. They utilized machine learning and time series analysis techniques, including regularization and PCA, to create robust portfolios of 70 stocks that outperformed 10-year U.S. Treasury Bonds by over 200% in risk-adjusted returns. Additionally, they built a pipeline from API to model, streaming 60 million stock data points (approximately 10 GB) from public APIs and developing financial metrics to ensure model validity and sustainability. The team also deployed a reinforcement learning pipeline with a Streamlit web app, simplifying user interactions and enabling portfolio visualizations for non-technical stakeholders and users.
Outschool
Our Team: Seong Youn (Amy) Cho
Faculty Mentor(s): Victor Palacios
Company Liaison(s): Olga Boldarieva, Christopher LeeThe team delivered an end-to-end project using SQL, Python, and dbt to productionize a groundbreaking dbt model in Amazon Redshift, creating predictive tags for purchase indicators expected to increase revenue by $2 million annually and improve user retention by 20%. As interns, they collaborated with Product, Engineering, and other teams in cross-functional workflows to provide data-driven insights and build predictive tools for company-wide business strategies. They utilized SQL and Python to query and analyze pricing data, providing sellers with accurate in-product pricing recommendations anticipated to increase bookings by $300K annually. Additionally, they designed and analyzed A/B testing results from pricing and retention experiments, launching email optimization and marketing strategies that led to a 30% increase in mobile app bookings.
Pendulum Intelligence
Our Team: Guarav Goyle, Yen Phan
Faculty Mentor(s): Robert Clements
Company Liaison(s): Tristin BeckmanThe team developed a model using KNN and LLM (RAG, FAISS) to match user scenarios with relevant narratives. They created a highly scalable data pipeline to scrape YouTube data using Regex and AWS Lambda, efficiently gathering 14 million data points and conducting in-depth analysis with Spark, resulting in a 90% reduction in processing times and a 22% cost reduction. They analyzed web engagement and user patterns to drive product enhancements and develop metrics for ongoing analysis. Additionally, they created nine unique data collection models for computer vision data curation, initiated data scraping, designed the architecture, and conducted processing and clustering analysis from over 100 sources. As interns, they performed ETL and quality control on customer interactions to identify usage patterns for feature engagement, product development, and future event tracking.
PG&E
Our Team: John Bailey (Elyse), Jessica Brungard (Carolyn), Indar Kumar, Fred Serfati
Faculty Mentor(s): Victor Palacios
Company Liaison(s): Audrey Cheon, Elyse Cheung-SuttonThe team queried data using SQL and conducted exploratory data analysis (EDA) with Pandas on over 5.5 million power outage records. They developed, fine-tuned, and deployed custom machine learning algorithms (Decision Trees, Random Forest, XGBoost) with Scikit-Learn to predict unplanned outage durations, achieving an 18% reduction in loss compared to previous models. Additionally, they enhanced model performance by 12% through hierarchical clustering and segmented modeling with PySpark. These improvements are expected to increase customer satisfaction by 30% through more accurate outage duration predictions. They communicated complex technical concepts, such as cluster analysis and ML models, to over 15 technical and non-technical stakeholders using clear data visualizations during monthly executive presentations, facilitating data-driven decisions.
San Francisco County Transportation Authority
Our Team: Yazhu Jiang, Dawei Pang
Faculty Mentor(s): Daniel Jerison
Company Liaison(s): Drew CooperThe team developed an automated pipeline that improved data processing efficiency by 50%, combining and cleaning approximately 250,000 rows of unstructured daily ridership predictions from various transit agencies using Python. They generated markdown and CSV files to compare observed and predicted data for model performance evaluation and validation. Additionally, they developed over 10 interactive dashboards using SimWrapper to quickly detect model errors such as ridership discrepancies.
Simplr, An Asurion Company
Our Team: Kabir Nawani, Arios Liang Tong
Faculty Mentor(s): Mustafa Hajij
Company Liaison(s): Ilias MiraouiThe team designed automated pipelines to generate preference datasets from over 92,000 chat histories for fine-tuning, ensuring high data quality. They performed Parameter-Efficient Fine-Tuning (LoRA) on the Mistral-7B model using 63,000 chat histories with the DPO algorithm and RLAIF on an A100 GPU, achieving a 21% increase in the ROUGE-L score. Additionally, they conducted Few-Shot Prompting to compare fine-tuned models to current ones in reasoning and summarization, using Llama-2 as the judge, and outperformed existing models in 70% of experimental trials.
SnapLogic
Our Team: Li En (Belinda) Ong, Justin Yang
Faculty Mentor(s): Mahesh Chaudhari
Company Liaison(s): Rachel Fournier, Hong Xu, Dr. Greg BensonThe team analyzed over 10 years of Salesforce and SnapLogic Platform data in BigQuery, revealing that pre-sales strategies increased average account annual recurring revenue (ARR) by $280k. They found insights leading to a 50% increase in purchases and reduced the time to the first add-on purchase by 30 days. They constructed predictive metrics to forecast ARR with an adjusted R² of 0.82, aiding the sales team in managing at-risk accounts and targeting growth opportunities. Additionally, they developed an upsell predictive model with an accuracy of 0.75 and an AUC of 0.82, which was deployed in a Looker Studio Dashboard.
Square (formerly Block Inc.)
Our Team: Vidith Balasa, Bassim Eledath
Faculty Mentor(s): Mahesh Chaudhari
Company Liaison(s): Aditi Sharma, Zixiao Huang, Yue YouThe team developed and trained a light gradient boosting model on a dataset over 100GB to predict local business lifetime value for advertising campaigns. They improved the lifetime revenue prediction pipeline's performance by more than 20% through the integration of Prefect 2 frameworks, which streamlined model deployment. Additionally, they secured shareholder approval for the model's production deployment by effectively presenting its financial benefits. They also co-developed an LLM RAG system that automated email campaign writing, significantly saving time for the marketing team.
Stanford Ophthalmic Informatics and Artificial Intelligence Group (OPTIMA)
Our Team: Rithvik Donnipadu, Maxim Sivolella
Faculty Mentor(s): Cody Carroll
Company Liaison(s): Dr. Sophia WangStudents led the development of a machine learning-based disease prediction model product to forecast glaucoma progression, aiming to reduce doctor workload and save approximately $600,000 annually. They analyzed 10 million rows of medical data, extracted over 500 features using BigQuery on GCP, and trained a custom multistage model. By incorporating functional PCA and a Random Forest regressor, they achieved a 34% improvement in RMSE compared to the baseline. Additionally, they designed a cohort of glaucoma patients using over 1 million data points and 1 terabyte of data, performing feature engineering to create 400+ predictors from Electronic Health Records, which enhanced model accuracy by 30%. Their work included constructing a global disease progression function using PCA and longitudinal data to forecast future vision loss, potentially saving over 700 patients from irreversible vision loss and $6 million in surgical fees annually.
SuperTech FT
Our Team: Yepeng Li, Kefeng Xiao
Faculty Mentor(s): Mustafa Hajij
Company Liaison(s): Dr. Albert Hu, Dr. Veera Nallam, George WilliamsThe team developed an AI-powered educational platform that leverages machine learning to analyze student performance and generate customized training materials. They engineered an ETL pipeline connecting cloud databases with local models, implementing low-latency data processing to deliver personalized content to users. The system includes automated problem set generation capabilities integrated with recommendation algorithms that draw from processed educational content. Through standardized ETL scripts optimized for both local and cloud environments, the team achieved significant operational efficiency improvements. The platform successfully served an initial user base, demonstrating positive learning outcomes and projected performance improvements in standardized testing. Students using the system benefited from personalized feedback loops and adaptive content delivery, supported by robust data collection and processing infrastructure.
The Nature Conservancy
Our Team: Ryan Bernstein, Seneth Waterman
Faculty Mentor(s): Cody Carroll
Company Liaison(s): Kirk Klausmeyer, Nathaniel RindlaubStudents developed an LLM pipeline to analyze over 1,000 pages of environmental policy, potentially saving The Nature Conservancy up to $400,000 in consulting fees. They fine-tuned a large language model on this data, enhancing summarization and question-answering capabilities, and documented their extensive experimentation in a research paper. Additionally, they created PyTorch-based object detection algorithms to classify species in over 30,000 wildlife images stored in AWS, and built an evaluation pipeline that improved summarization accuracy by 20%. In another project, they automated workflows with a custom ETL pipeline, achieving a 90% reduction in manual work and providing real-time groundwater depth monitoring with interactive dashboards.
TruckX Inc
Our Team: Solomon Asiedu-Ofei, Princewell Egbujor, Shrey Jain, Param Mehta, Lance Santerre
Faculty Mentor(s): Victor Palacios
Company Liaison(s): Clarissa DanilovStudents developed a Python-based truck stop recommendation system to help drivers plan their journeys efficiently and managed the core codebase with version control. They also created a unified battery degradation model with 70% accuracy for early failure detection in lithium-ion and solar batteries. The team spearheaded GitHub integration for version control, significantly improving the software development lifecycle by establishing a structured workflow. They engineered a predictive model with over 90% accuracy for detecting sharp turn maneuvers from extensive driving data, and are working on a geofence recommendation feature using unsupervised clustering to enhance truck geofencing. Additionally, they built and deployed an intelligent geofence recommender feature that increased client-app engagement by 200% and automated 80% of geofence implementation. An AI-powered Q/A chatbot was developed using GPT-4, RAG, Langchain, and Streamlit to improve trucking compliance and document accessibility. The team also engineered a rule-based sharp turn detection algorithm and geospatial predictive features for truck behavior analysis.
Manor Lab - University of California, San Diego
Our Team: Jeffrey Chen, Abhijeeth Erra
Faculty Mentor(s): Cody Carroll
Company Liaison(s): Dr. Uri ManorThe team developed and published an open-source deep learning-based GUI that allowed researchers to visualize and conduct exploratory and quantitative analysis of auditory brainstem response (ABR) waveforms, providing essential resources for data analysis. They trained and cross-validated a Convolutional Neural Network (CNN) using PyTorch, achieving 94% accuracy in classifying ABR waves as signal or noise, which was competitive with the benchmark models for ABR thresholding. Additionally, they trained and cross-validated a two-step method for detecting the peaks and troughs of ABR waveforms using another CNN and tools from Scikit-Learn, resulting in a 0.1 ms error for Wave I Latency, surpassing industry standards.
UCSF - Oncology
Our Team: Shreyas Anil, Bhumika Srinivas
Faculty Mentor(s): Yannet Interian
Company Liaison(s): Dr. Hui LinThe team processed over 12 million words of clinical notes and unstructured EHR data for cancer patients, achieving a 25% reduction in note volumes. They enhanced early disease detection for more than 5,000 patients by developing hierarchical segmentation pipelines with encoder-driven transformer models, resulting in an 8% accuracy improvement through XGBoost. Fine-tuned embedding models like GritLM and GIST, combined with Mistral-7B and LLaMA architectures, were applied to refine glioma patient note clustering and expand vocabulary. At the AAPM Conference, the team, as first authors, demonstrated that while baseline MPT-7B and ClinicalBERT models did not inherently improve EHR notes' predictive accuracy, fine-tuning ClinicalBERT achieved F1 scores between 0.81 and 0.91, AUROC between 0.79 and 0.87, and a 6% accuracy improvement over the baseline. Additionally, they led a study to boost LLM performance for classification tasks in healthcare, developed a pipeline for identifying high-risk patients using fine-tuned open-source LLMs, and processed clinical text to create templated summaries using GPT-4. Their retrieval-augmented generation (RAG) framework, which used kNN clustering on patient embeddings, improved classification accuracy and F1 scores by 25% on a testing set of 500 samples, competing with fine-tuned ClinicalBERT metrics. They also experimented with long-context tuned versions of MPT-7B, LLaMA-2, and LLaMA-3, focusing on sequence parallelism and quantization for multi-GPU inference. The abstract was selected as "Best in Physics" for the AAPM conference in July 2024.
UCSF - Clinical Informatics
Our Team: Chris Nishimura, Yi-Fang Tsai
Faculty Mentor(s): Shan Wang
Company Liaison(s): Dr. Xinran LiuThe team utilized SparkSQL to process 1.5 million rows of unstructured and structured data from UCSF, including medical progress and case management notes, daily vital signs, and lab results for 150,000 patient encounters. They enhanced hospital throughput planning by developing predictive models for patient length of stay, employing PyTorch and Transformer libraries to fine-tune a pre-trained UCSF BERT medical LLM with unstructured note data. By implementing a stacking technique, they combined the UCSF BERT NLP model with classical machine learning models trained on structured data, improving the predictive performance of their multimodal model outputs. They also engaged with the UCSF Director of Clinical Informatics to align research efforts with institutional needs and present their findings.
UCSF - Gastroenterology
Our Team: Eren Bardak, Yihan Cao
Faculty Mentor(s): Shan Wang
Company Liaison(s): Dr. Vivek RudrapatnaThe team utilized models like Lasso/Ridge Regressions, Random Forest, and XGBoost to predict overall patient states, achieving 80% accuracy through cross-validation and hyperparameter tuning. They conducted visual analyses on drug efficacy using Python visualization packages such as Matplotlib and Seaborn, and proposed potential medication actions for reinforcement learning agents. Additionally, they developed and deployed a Python package via PyPI to facilitate enhanced clinical data interpretation. They queried and analyzed over 100 GB of confidential medical data using SQL on Azure Data Studio, adhering to data privacy standards. The team also implemented offline reinforcement learning algorithms, compared RL-identified policies with real-world decision-making, and conducted extensive data analysis and quality checks. They are currently working on models like the Decision Transformer for reinforcement learning through sequence modeling.
UCSF - MoonLAIT Lab
Our Team: Eric Shen, Kejia Wang, Claire Zhou
Faculty Mentor(s): Shan Wang
Company Liaison(s): Dr. Yue LengThe team designed a process to handle multidimensional, multimodal polysomnography (PSG) and 676 GB of health data from over 70,000 observations in large-scale cohort studies for Parkinson’s disease diagnosis. They enhanced predictive power by 50% through feature extraction and the application of machine learning models like logistic regression, random forest, and XGBoost. The team addressed imbalanced datasets using oversampling (SMOTE) and undersampling (Tomek Links), tuning hyperparameters through cross-validation and evaluating model performance with metrics such as R-squared, AUROC, and AUPRC. They developed algorithms to engineer biological features from 1,400 GB of raw PSG data and established correlations between biomarkers and neurodegenerative diseases using statistical analysis.
University of London, Royal Holloway
Our Team: Mikkel Ovesen
Faculty Mentor(s): Yannet Interian
Company Liaison(s): Sara BernardiniThe team developed advanced Reinforcement Learning techniques to optimize combinatorial algorithms for Boolean satisfiability problems (SAT) and multi-agent path finding (MAPF). They enhanced the learning capabilities of their RL model by implementing a modified Actor-Critic approach with eligibility tracing, resulting in approximately a 57% reduction in epochs to reach the previous best score. Additionally, they innovated the integration of Experience Replay in the REINFORCE framework, achieving around a 30% increase in computational speed by leveraging past experiences to improve algorithmic performance.
Upwork
Our Team: Shagun Kala, Aditya Nair
Faculty Mentor(s): Yannet Interian
Company Liaison(s): Adam RhubergThe team analyzed over 200 million rows of data on Snowflake using Apache Spark in Databricks to derive insights from client and freelancer interactions. They developed a churn definition that captured 92% of diverse client behaviors and engineered predictive features through exploratory data analysis. Their innovative ML framework included Survival Analysis and Decision Tree classifiers to predict client churn, and they productionized the model. Additionally, they designed A/B tests and improved client retention by 8% through a tailored marketing strategy utilizing a fine-tuned large language model.
US Department of Health and Human Services
Our Team: Caleb Hamblen, Nicholas Miller
Faculty Mentor(s): Yannet Interian
Company Liaison(s): William KimThe team implemented a data acquisition system using Selenium to scrape thousands of podcast episode records, automating data aggregation and saving the delivery team 12 hours per week. They deployed Airflow DAGs to schedule automated scraping jobs, storing the results in AWS S3 and creating interactive Plotly graphs hosted on Domo for centralized data access. Additionally, they used the OpenAI API for sentiment analysis on news article comments, providing valuable insights into public feedback on health campaigns managed by the Office of the Surgeon General.
Vibrant Data Labs
Our Team: Tatshini Ganesan, Ting Pan
Faculty Mentor(s): Yannet Interian
Company Liaison(s): Lara Reichmann, Eric Berlow, Rich WilliamsThe team improved user engagement by 5% through the implementation of Google Analytics tracking for an embedded player on the website. They developed a classification model to categorize organizations into four groups based on descriptions for updating an interactive map tool. This involved using an LLM to generate embeddings from descriptions and applying a KNN model as a baseline. By fine-tuning Flan-T5 and ClimateBert LLM models with PyTorch, they achieved a 31% increase in accuracy.
-
AGMonitor
Student Team: Chenxi Li, Theodore Mefford
Faculty Mentor(s): Shan Wang
Company Liaison(s): Stanley Knutsen, Dr. Tim HartzProject Outcomes: The "Crop Alert to Protect Farms and Save Water" project aimed to decrease water usage during droughts while preserving crop yields and quality. Utilizing AgMonitor's vast data resources, students developed and validated water stress and soil moisture predictors. This environmentally beneficial initiative impacted agriculture's water consumption, benefiting 200,000 acres in California and utilizing the expansive OpenET dataset across 14 states.
Alaska Airlines
Student Team: Joren James, Haonan Li, Anirav Jain
Faculty Mentor(s): Shan Wang
Company Liaison(s): Tak WongProject Outcomes: In two innovative projects, students endeavored to elevate Alaska Airlines' marketing approach and enhance the guest experience. Project 1 focused on refining the promotion of the Mileage Plan program and the Alaska Airlines Visa Signature Card. Through meticulous data analysis, students pinpointed optimal moments for marketing, considering guest interactions, flight frequency, geographical relevance, and signup likelihood. This strategic approach maximized the impact of marketing efforts. Project 2 delved into audience segmentation, uncovering diverse guest preferences, from fare-conscious travelers to those seeking amenities. Tailored promotions aligned with distinct guest segments, improving the overall Alaska Airlines experience.
AWS
Student Team: Adit Shrimal, Kuan Pin Chen, Maneel Karri, Ajayeswar Peddyreddy
Faculty Mentor(s): Robert Clements
Company Liaison(s): Brad Kenstler, Anila Joshi, Vidya Sagar Ravipati, Divya BhargaviProject Outcomes: MLSL enlisted students to develop modular ML solutions for targeted industries (healthcare life sciences, media & entertainment, manufacturing). Their goals included collaborating with MLSL's repeatable solutions team on various projects, spanning multi-modal solutions, computer vision, forecasting, and knowledge graph modeling, addressing specific industry needs and challenges.
Atlassian
Student Team: Johnny Ka Chun Chau, Yuan Yao
Faculty Mentor(s): Robert Clements
Company Liaison(s): Chayan ChakrabartiProject Outcomes: In this project, students were tasked with using machine learning to build prototype features designed to enhance user productivity and satisfaction. Students worked on various ML models, including deep learning and gradient boosted trees, experimenting with new approaches. They also played a role in designing advanced features and embeddings, evaluated model performance, and collaborated closely with experienced machine learning scientists, engineers, and data scientists to contribute to prototype platform features.
BlackRock
Student Team: Amy Tang, Theo Byunghyn Kim
Faculty Mentor(s): Jeff Hamrick
Company Liaison(s): Srividya Krithivasan, Victor MoraProject Outcomes: Students collaborated with internal data science teams to create a Finance Chatbot. The project aimed to enhance sales analytics by employing NLP/AI technology for query responses. They explored various NLP algorithms and datasets, concluding with creative visualizations for stakeholder communication and successful deployment within the firm's infrastructure.
Blueboard
Student Team: Matt Marwedel, Jazz Sun
Faculty Mentor(s): Robert Clements
Company Liaison(s): Michael Su, Jason WeinerProject Outcomes: Students undertook a project involving NLP analysis of client feedback surveys. Their goal was to extract features from unstructured feedback and create a localized model to differentiate between experience provider-related issues, concierge-related issues, and external problems. Additionally, they worked on data ETL, focusing on transitioning ETL processes from cloud-based no-code tools to an Airflow-based pipeline for tools like Zendesk and Salesforce. They also planned a data mart exercise to determine tables for prosumer usage, serving COO, engineering, data analysts, and others.
Boston Children’s Hospital
Student Team: Yu-Chuan Chiu, Deepak Singh
Faculty Mentor(s): William Bosl
Company Liaison(s): Michelle Bosquet Enlow, PhDProject Outcomes: Students engaged in a project titled "supervised tensor and matrix joint factorization for multimodal data fusion and biomarker extraction." They utilized Python, tensor and matrix factorization, Bayesian statistics, and machine learning to analyze EEG data for early prediction of mental and neurodevelopmental disorders. Their computational objective was to develop a coupled tensor and matrix factorization algorithm (SupCP+M) and apply it to a neurodevelopmental dataset containing EEG, clinical measures, sociodemographic indicators, and genetic data. The project aimed to extract interpretable nonlinear EEG features as potential biomarkers for brain-based disorders, with a focus on childhood anxiety and cognitive neurodevelopment. Students also worked on graphical representations of latent features and offered opportunities for learning in nonlinear dynamical analysis and computational neuroscience.
Buck Institute
Student Team: Lingraj Vannur
Faculty Mentor(s): Daniel O’Connor
Company Liaison(s): Chunkai Zhou, PhDProject Outcomes: Students in the Zhou lab developed a deep learning-based imaging analysis platform to map aging-related protein changes in cells, aiming to create an aging molecular roadmap. Using Python, Java, and TensorFlow, they enhanced existing neural networks and streamlined data analysis while co-authoring research papers. In the second project, they explored the potential of Alphafold2 and molecular dynamics simulations to predict protein folding and assist drug/antibody selection, contributing to structural biology advancements with machine learning tools.
California Department of Fish and Wildlife
Student Team: Xin Ai, Sharon Dodda
Faculty Mentor(s): James Wilson
Company Liaison(s): Alex Heeren, Brett FurnasProject Outcomes: Students at the Wildlife Health Laboratory (WHL) in collaboration with CDFW scientists focused on resolving human-wildlife conflicts, particularly with black bears. Their research aimed to update the state's black bear conservation plan. Using text and sentiment analysis, they examined social media data from platforms like Twitter and Nextdoor, expanding previous work on coyotes. Students aimed to identify patterns in black bear discussions and develop a real-time data dashboard for wildlife monitoring.
Candid
Student Team: Zemin Cai, Harrison Jinglun Yu
Faculty Mentor(s): Shan Wang
Company Liaison(s): Cathleen ClerkinProject Outcomes: Candid's Insights department engaged students in impactful research projects in data ethics. These projects included an examination of diversity, equity, and inclusion within nonprofits, an exploration of nonprofits' societal impact, and an investigation into real-time grantmaking data, particularly in relation to issues like racial equity. Students were tasked with identifying factors influencing organizations' willingness to share demographic data and analyzing data to predict nonprofits' societal impact. Additionally, they explored methodologies to provide real-time insights into philanthropic trends while addressing potential biases and confounding factors. These projects harnessed various data science techniques and underscored the importance of ethical considerations in data analysis.
Carbon Health
Student Team: Guru Gopalakrish
Faculty Mentor(s): Mustafa Hajij
Company Liaison(s): Hoda NoorianProject Outcomes: This project addressed predicting no-show appointments in urgent care, researched industry best practices, and built a model MVP. They also sought to personalize appointment reason lists based on user data, leveraging Recommendation Systems, with potential production implementation and impact analysis on appointment conversions.
Dagshub
Student Team: Kang-Chi Ho, Yichen Zhao
Faculty Mentor(s): Robert Clements
Company Liaison(s): Nir Barazida, Guy Smoilovsky, Dean PlebanProject Outcomes: Students involved in these projects undertook a wide range of tasks and initiatives. In the first project, they delved into the integration of machine learning tools with DagsHub, fostering innovation through novel integrations and content creation. The second project centered around replicating and expanding upon Chinchilla's research, involving the tracking of components and a comprehensive review of prior work, all aimed at increasing the accessibility of Large Language Models. Lastly, in the third project, students took part in extending a HackerNews bot's functionalities. This extension allowed for user input regarding content preferences and the development of a recommendation engine, with the ultimate goal of delivering valuable contributions to the technology community.
Environmental Defense Fund
Student Team: Varun Hande, Adam Ansari
Faculty Mentor(s): Mustafa Hajij
Company Liaison(s): Christopher CusackProject Outcomes: Students improved fishery monitoring by enhancing ML algorithms for SmartPass, a smart camera system. The aim was to democratize AI algorithms, making them accessible to more practitioners and boost global fisheries management.
Fitbod
Student Team: Akshay Pamnani, Patricia Ornelas
Faculty Mentor(s): Victor Palacios
Company Liaison(s): Thiago Marzagão, Esther LiuProject Outcomes: Students utilized Python, SQL (with Google Big Query), basic statistics (mostly hypothesis testing), machine learning, and Tableau. In the first project, they improved calorie burn estimation for more accurate user tracking and better recommendations. In the second project, machine learning helped predict workout duration, optimizing exercise recommendations.
Four Analytics
Student Team: Ensun Park, Nischal Mishra
Faculty Mentor(s): Jeff Hamrick
Company Liaison(s): Kirby ZhangProject Outcomes: Students aimed to enhance a pricing system based on labor hours. They considered factors like client history, scope, location, and space size. In cases with ample historical data, they sought a real-time ML model, incorporating market rates, square footage, days, etc., to align prices with client expectations. They were also tasked with using clustering techniques for cases with less historical data.
W.L. Gore & Associates
Student Team: Cho Hsum Yang, Camilo Chaves Atlassian
Faculty Mentor(s): Daniel O’Connor
Company Liaison(s): Vasu Venkateshwaran, Noah Hodgson, James CroninProject Outcomes: Students worked with image data from microscopy and pathology experiments at Gore, aiming to relate material structure to properties. They utilized ML and computer vision techniques for semantic/panoptic segmentation, boundary/key point detection, and practical metric extraction. They also explored data augmentation and synthetic generation. Finally, they developed user-friendly ML model training and usage code within an existing Python library.
Kidas Inc.
Student Team: Raghavendra Kommavarapu
Faculty Mentor(s): Mustafa Hajij
Company Liaison(s): Amit YungmanProject Outcomes: Students optimized point-of-interest detection algorithms, including hate speech and sexual content detection, using data and metadata. They took part in developing age detection in audio and text, emotion detection in audio and text, and voice changer detection in audio. Additionally, they worked on displaying data visualizations on personal pages based on user activity and algorithm results using Python.
KNIME
Student Team: Jinwei Sun
Faculty Mentor(s): Victor Palacios
Company Liaison(s): Victor PalaciosProject Outcomes: The student team learned KNIME and Pytorch focusing graph neural networks. They produced business-oriented articles and videos showcasing tool usage, gaining skills for explaining deep learning to non-technical audiences. This role also involved teaching KNIME in paid courses, emphasizing the intersection of education and data science, including public speaking and business engagement.
Metaphor Data
Student Team: Aydin Schwartz, Prithvi Nuthanakalva
Faculty Mentor(s): Diane Woodbridge
Company Liaison(s): Kirit Basu, Mars LanProject Outcomes: The team has developed a Q&A Slack/Teams bot using OpenAI's ChatGPT LLM to answer natural language questions related to customer's datasets, dashboards, and knowledge base. They have also added a Generative AI feature to summarize long Slack threads into digestible knowledge that can be persisted for future references. Both features have since then been rolled out to customers for testing.
Metropolitan Transportation Commission
Student Team: Akul Bajaj, Lantin Su
Faculty Mentor(s): Cody Carroll
Company Liaison(s): Kearey Smith, Kaya Tollas, Aksel OlsenProject Outcomes: Students undertook four projects for the Metropolitan Transportation Commission (MTC), encompassing data engineering, machine learning, and data analysis. Their primary objective was to automate data processes, enhance data accuracy, and facilitate informed decision-making. These projects involved diverse tools and techniques such as Python, AWS, natural language processing, data visualization, image classification, and machine learning. The students contributed to improving regional planning, resilience evaluation, data management, and predictive modeling within MTC, aligning with the organization's mission to enhance transportation infrastructure and resilience.
Oportun Inc.
Student Team: Hanna Siew Tsien Lee, Shubhangi Badwaik
Faculty Mentor(s): Jeff Hamrick
Company Liaison(s): Jonathan SageProject Outcomes: Students used Python, SQL, AWS Cloud, and machine learning in two projects. The first, "Member re-engagement Propensity Modeling," aimed to understand customer behavior and engagement across Oportun's ecosystem, enabling better personalization. Techniques included graph analysis and building a re-engagement propensity model. The second project involved migrating Credit Card/Embedded Finance to a containerized infrastructure, enhancing workflow and reducing costs while providing hands-on experience with modern data infrastructure.
Pendulum
Student Team: Kyle Kayhan Eryilmaz, Youshi Zhang
Faculty Mentor(s): Daniel Jerison
Company Liaison(s): Tristin BeckmanProject Outcomes: Students collected video transcripts and metadata from various media platforms, employing pretrained language models like BERT, RoBERTa, and BART for sentiment analysis, topic modeling, entity recognition, and narrative detection. They utilized SQL and Python for data extraction and analysis, and employed frameworks like HuggingFace, PyTorch, Sci-kit learn, and Metaflow, alongside AWS, for model training and deployment. Their projects aimed to identify influential content creators and extract interview details from video content, enhancing understanding of content dissemination and creator communities.
PG&E
Student Team: Matthew Wheeler, Nhi Pham Nguyen
Faculty Mentor(s): Jeff Hamrick
Company Liaison(s): Michael SignorottiProject Outcomes: Students worked on the Image Labeling Infrastructure Development project. They aimed to streamline the collection, quality control, and utilization of labeled data for the computer vision team. They enhanced existing code, created labeling and quality control scripts, and planned to migrate this to a workflow execution tool. Tools such as SageMaker, GroundTruth, Jenkins, Jupyter Lab, GitHub, and Python were utilized.
Propeller Health
Student Team: Preetham Pathi, Manish Vuppugandla
Faculty Mentor(s): Shan Wang
Company Liaison(s): Connelly Doan, Noah MatsuyoshiProject Outcomes: The students' project at Propeller focused on deriving insights from behavioral analytics data related to respiratory disease patients using the mobile app. They constructed a Patient Experience Product Metrics Tableau workbook, delving into app behavior data and exploring creative ways to display and analyze metrics. They also conducted exploratory modeling to understand the relationship between app engagement and patient retention, providing direction for patient engagement strategies. Technologies included Redshift (SQL) for reporting queries and Python/Amazon Sagemaker for modeling.
Salk Institute
Student Team: Yu-Hsin Wang, Mohana Medisetty
Faculty Mentor(s): Cody Carroll
Company Liaison(s): Uri ManorProject Outcomes: The students engaged in projects at the WABC involving vast image datasets from various sample types, including brain, tumor, and plant tissues. They leveraged Python-based libraries for deep learning, addressing tasks such as disease state prediction, developing a deep learning-based image degradation tool, object tracking in live cell videomicroscopy data, and motion prediction from single snapshots. Additionally, they explored new loss functions for super-resolution to enhance image quality. The goal was to streamline these tasks into accessible pipelines like imjoy or napari.
San Francisco County Transportation Agency
Student Team: Pei Wang, Madhav Ponnudurai
Faculty Mentor(s): James Wilson
Company Liaison(s): Dan TischlerProject Outcomes: The students worked on three projects for SFCTA. Project #1 involved building a public-facing count portal to facilitate identification and dissemination of vehicle, pedestrian, and bicycle counts collected over a decade. Project #2 utilized the SimWrapper platform to create dashboards reporting travel demand forecasting model outputs and facilitating scenario comparisons. Project #3 focused on developing methods to enhance SimWrapper's capacity to display large skim datasets for better QA/QC and analysis of transportation network changes.
SoFi Stadium
Student Team: Ity Soni, Justin Can
Faculty Mentor(s): Daniel Jerison
Company Liaison(s): Melanie PalmerProject Outcomes: The students contributed to the Data Strategy team at SoFi Stadium and Hollywood Park, utilizing Google Analytics Suite, Python, R, and machine learning techniques. They worked on three projects: creating an internal pricing tool for events, conducting consumer market basket analysis to optimize marketing strategies, and performing sentiment analysis on event surveys to identify guest pain points and improve operational workflows. These projects aimed to enhance revenue generation and customer experience.
Stanford Graduate School of Business
Student Team: Rushil Manglik
Faculty Mentor(s): Victor Palacios
Company Liaison(s): Natalya Rapstine, Amy NgProject Outcomes: The students engaged in a project called "Layout Parser" at the GSB, where they tackled challenges related to parsing table text or numbers from old documents, some dating back to pre-1900. They explored deep learning approaches using modern layout parsers to automate the extraction of information from tables with varying layouts. The goal was to improve accuracy and efficiency when dealing with old or misformatted tables, where manual transcription was time-consuming and costly.
Stanford University, Ophthalmic Informatics and Artificial Intelligence Group
Student Team: Vichitra Kumar, Devendra Govil
Faculty Mentor(s): Cody Carroll
Company Liaison(s): Sophia WangProject Outcomes: Students explored the integration of various data modalities, including electronic health records, free-text data, and ophthalmic patient images, to create predictive models for glaucoma progression. They also worked on enhancing model trustworthiness by developing approaches for explaining complex clinical prediction models that use multiple data modalities, such as structured data, text data, and imaging data from electronic health records.
Subwire
Student Team: Bharadwaj Allu, Harsh Praharaj
Faculty Mentor(s): Mustafa Hajij
Company Liaison(s): Michael Terry, Alex DavidoffProject Outcomes: The students worked on two projects within the context of SubWire. One project involved creating a model to collect and analyze user behavior metrics on the SubWire app, including watch time, shares, and their impact on user retention. The other project utilized web scraping techniques to gather user data from various social media platforms, aiming to develop a predictive model for virality based on relationships and engagement metrics.
Target
Student Team: Tejashree Ladhake, Akhil Gopi, Abhradeep Mukherjee
Faculty Mentor(s): Diane Woodbridge
Company Liaison(s): Joey AhnnProject Outcomes: The students designed and developed algorithms for generating complementary recipes based on user-entered recipes. They created an automated and scalable data pipeline that collects recipe and review data from various sources. This data was then integrated with a neural network-based flavor graph to calculate candidate recipes that pair well with the user's input. The resulting output takes into account both complementarity and diversity to enhance the overall user experience.
The Nature Conservancy
Student Team: Wan Chun Liao, Jessica Xinyi Wang
Faculty Mentor(s): Cody Carroll
Company Liaison(s): Kirk Klausmeyer, Nathaniel RindlaubProject Outcomes: Students collaborated with The Nature Conservancy's Conservation Technology team, contributing to environmental conservation through data science. In Project 1, they developed a data pipeline to estimate flooding extent on fields used to support migratory wetland birds. In Project 2, they refined a wireless camera trap system using machine learning to identify invasive species and protect endemic wildlife on islands, focusing on Santa Cruz Island off California's coast. Their work helped enhance monitoring and conservation efforts.
University of California, San Francisco: Clinical Informatics
Student Team: Ankit Gupta, Joy Chuyi Huang
Faculty Mentor(s): Shan Wang
Company Liaison(s): Xinran Liu, MD, MS, FAMIAProject Outcomes: Students at UCSF collaborated on two projects. In the first project, they aimed to revolutionize physician evaluation metrics, similar to how sabermetrics transformed baseball. They explored various data science techniques, from traditional statistics to NLP, to assess physician discharge effectiveness. In the second project, students worked on predicting acute postpartum care utilization to reduce maternal morbidity. They refined an existing model using clinical data and machine learning, ultimately striving to optimize outpatient postpartum visits. Their work aimed to enhance healthcare practices and patient outcomes.
University of California, San Francisco: Gastroenterology
Student Team: Daniel Tinoco, Tzu An Wang
Faculty Mentor(s): Shan Wang
Company Liaison(s): Vivek RudrapatnaProject Outcomes: Students contributed to two projects. In the first project, they aimed to assess the environmental and economic implications of different colon cancer screening methods. They used Markov modeling and Bayesian methods to estimate carbon emissions associated with screening options, potentially influencing healthcare decisions and policy. In the second project, students worked on information extraction from clinical notes to enhance patient-level prediction modeling using electronic health records. Their contributions supported the development of algorithms for transforming unstructured clinical data into analyzable formats, improving patient care.
University of California, San Francisco: Oncology (NLP)
Student Team: Max Yizhi Ma, Sanchita Jain
Faculty Mentor(s): Carlos Garcia
Company Liaison(s): Dr. Hui Lin, Dr. Jorge BarriosProject Outcomes: Students participated in a project focused on developing Natural Language Processing (NLP) transformer models for estimating the prognosis of cancer patients using Electronic Health Record (EHR) clinical notes. They utilized various transformer models, including ClinicalBERT and XLNet, to analyze over 160,000 oncology data registries collected over a decade. The project aimed to enhance cancer care by predicting overall survival across multiple cancer sites and provided valuable experience in NLP and data mining in the medical field.
University of California, San Francisco: Oncology (CV)
Student Team: Andres Martinez, Riley Tianrui Hu, Yusong Wang
Faculty Mentor(s): Carlos Garcia
Company Liaison(s): Dr. Tomi Nano, Dr. Hui Lin, Dr. Dante CapaldiProject Outcomes: Students participated in a project focused on automating the identification and segmentation of brain lesions in magnetic resonance (MR) images for radiosurgery. They utilized deep learning techniques with PyTorch, working with 3D MR images. The project aimed to enhance efficiency in radiosurgery treatment workflows, with guidance from experienced medical physicists.
YLabs (Youth Development Labs)
Student Team: Tejaswi Dasari
Faculty Mentor(s): Diane Woodbridge
Company Liaison(s): Robert OnProject Outcomes: Students in the CyberRwanda project used various technologies and techniques to measure project progress and effectiveness. They employed Google Analytics to track engagement metrics and designed KPI dashboards for automatic data generation. However, challenges included manual data tracking, discrepancies between Google Analytics versions, and gaps in tracking product pick-ups. Integrating and utilizing data from different sources including MongoDB pharmacy backend for decision-making was identified as a crucial goal. In addition, the students developed an automated chatbot that can generate answers using natural language processing and existing documents, reducing the wait time.
-
ACLU
Our Team: Joleena Marshall
Faculty Mentor(s): Michael Ruddy
Company Liaison(s): Linnea Nelson, Tedde Simon, Brandon GreeneProject Outcomes: The team developed a tool with Python to acquire and preprocess publicly-available data related to the Oakland Unified School District to investigate whether or not OUSD’s allocation of resources results in inequities between schools. The team also provided an updated data analysis on educational outcomes for indigenous students for a select number of Humboldt County unified school districts, including data visualizations.
Bay Area Rapid Transit (BART)
Our Team: Zihao Ren, Yunhe Jia, Zipeng Hong
Faculty Mentor(s): Steve Devlin
Company Liaison(s): Wendy Wheeler, Yu Shen, Herbert DiamantProject Outcomes: The team implemented an analysis of BART train location data and location-related station message announcements across multiple data sources and tables within the BART system. The project began with exploratory data analysis to pinpoint and diagnose issues such mismatched location and messaging information for a given train, identification of error prone lines and stations, and lines or trains exhibiting unusually variable arrival times. The team then identified and fixed data engineering issues that often lead to problems, and built out statistical models to predict and quickly identify errors as they occur. Finally, the team built out an extract/transform/load (ETL) pipeline and train movement dashboard for identifying and communicating estimated time of arrival issues for trains.
BlackRock
Our Team: Abdus Khan, Isabella Zhai
Faculty Mentor(s): Jeff Hamrick
Company Liaison(s): Victor MoraProject Outcomes: The team developed a data-driven forecasting system for exchange-traded fund (ETF) flows. The team performed feature importance analysis to identify market and macroeconomic factors affecting the flows and experimented with different machine learning models to generate the forecasts. The team also provided a sensitivity analysis interpretation of how each market and macro-economic factor impacts ETF flows.
Blueboard
Our Team: Xinming Wang, Yufeng Xing
Faculty Mentor(s): Diane Woodbridge
Company Liaison(s): Michael Su, Taylor SmithProject Outcomes: The team developed a natural language processing (NLP) model to perform sentiment analysis on customer reviews. It also developed and maintained Airflow pipelines for data management purposes.
Boost
Our Team: Marti Heit
Faculty Mentor(s): Steve Devlin
Company Liaison(s): Mustafa Abdul-Hamid, Christian Hanish, Jorge CostaProject Outcomes: The team worked on a series of small projects including: probabilistic predictions of professional soccer matches in the English Premier League (EPL); clustering of NCAA basketball players based on their style of play; translation of player clusters into context-relevant skill sets; building a pipeline to automatically generate visualizations of shooting efficiency per shot zone in NCAA basketball; building a metric to quantify and predict game excitement in different sports; auto-generation of NCAA game reports with relevant match recap data and insights obtained using techniques from natural language processing.
California Department of Fisheries and Wildlife
Our Team: Chandan Nayak, Isaac Lo
Faculty Mentor(s): Brett Furnas, Christina SloopProject Outcomes: The team used machine learning and natural language processing (NLP) techniques to better understand human-wildlife intersection using social media data (e.g., by scraping Twitter).
California Forward
Our Team: Evie Klaassen
Faculty Mentor(s): Michael Ruddy
Company Liaison(s): Patrick AtwaterProject Outcomes: The team built a tool with Python to determine where high wage jobs are located in California. This tool serves as an extension to current data tools created and maintained by the organization. The team also developed a pipeline to clean and prepare new public data when it is released, and for the tool’s outputs to be regularly updated given any new data.
Cerenetics
Our Team: Rachit Yadav, Cameron Meziere
Faculty Mentor(s): James Wilson
Company Liaison(s): Skyler CranmerProject Outcomes: The team applied various statistical methods, as well as neural network models, to detect the presence of mental illness using fMRI (functional magnetic resonance imaging) data.
Environmental Defense Fund
Our Team: Ankush Gupta
Faculty Mentor(s): Michael Ruddy
Company Liaison(s): Christopher CusackProject Outcomes: The team worked on a computer vision project aimed at enhancing an object detection system in collaboration with CVision.ai. The team developed an object detection model that detects small fishery vessels entering and leaving a port with high precision and high inference speed, even in harsh weather conditions. In addition, the team developed a tool to automate the preprocessing step of converting a custom dataset to an object detection dataset format – saving manual efforts by the annotation team.
Facebook
Our Team: Edith Lee, Mateen Saifyan
Faculty Mentor(s): Yannet Interian
Company Liaison(s): Claire Broad, Anne Chittum, Mike FaheyProject Outcomes: Students built a daily landing extract/transform/load pipeline to query and aggregate internal pipeline metadata to assist in pipeline ownership assignment and pipeline deprecation. The team then designed and built a drill-down dashboard to effectively visualize the granularity of the generated data. Other tasks addressed by the team included updating existing data pipelines to meet current coding standards and constructing metrics to evaluate pipelines.
First Republic Bank
Our Team: Ronica Gupta, Arman Hashemizadeh
Faculty Mentor(s): Jeff Hamrick
Company Liaison(s): Aaron Frank, Xu Liu, Chris Csiszar, Mark WoodworthProject Outcomes: Embedded within the financial planning and analysis unit, the team used natural language processing (NLP) to solve their named entity recognition (NER) problem. We developed an end-to-end machine learning pipeline using NLP techniques, Bidirectional Encoder Representations from Transformers (BERT), and tree-based models to extract relevant information from 200-page-long portable document format (PDF) files.
Freedom Financial Network
Our Team: Jaysen Shi, Surbhi Prasad
Faculty Mentor(s): Jeff Hamrick
Company Liaison(s): James OlnessProject Outcomes: The team built a price optimizer model to recommend best loan rates, with the aim of maximizing the total number of loans provided by the company. The data was queried and organized using BigQuery from GoogleCloud Storage. The model was created using machine learning and optimization techniques in Python. The proposed loan rates replaced the recommendations of a third-party analytical partner after improvement was demonstrated in funded loans with the new model.
Golden State Warriors
Our Team: David Lyu, Britta Goldman
Faculty Mentor(s): Steve Devlin
Company Liaison(s): Ray YockeProject Outcomes: The team focused on combining disparate data sources, including Warriors internal data from summer camp enrollment, season ticket purchases, and Chase center retail sales, with external data from Ticketmaster and third-party ticketing apps. Once combined and cleaned, the team built a model to predict future purchases from past purchase history over various time frames. Finally, the team worked on streamlining and productionalizing the model with the engineering team, and interpreting actionable results with the marketing team.
Hims and Hers
Our Team: Karishma Chauhan, Jason Yu
Faculty Mentor(s): Diane Woodbridge
Company Liaison(s): Yao Liu, Long NguyenProject Outcomes: The team developed and productized time series models to predict the impacts of television advertisements. Additionally, the team developed and productized machine learning and deep learning models to predict customer lifetime value.
Metromile
Our Team: Kooha Kwon, Srividya Krithivasan
Faculty Mentor(s): Michael Ruddy
Company Liaison(s): Edwin Zhang, Colleen Qiu, Christopher Olley, Lindsay OrrProject Outcomes: The team improved a risk prediction model that estimates the total loss each policy will claim through feature engineering, hyperparameter tuning, and experimentation with pre-processing methods. In addition, the team also developed a new model that identifies the precise location of a street-parked vehicle and alerts the mobile app user of upcoming parking restrictions, such as street sweeping.
New York Mets
Our Team: Brendan Jenkins, Seungju Han
Faculty Mentor(s): Daniel Jerison
Company Liaison(s): Jake TofflerProject Outcomes: In baseball, the fielding team wants to know where the ball is likely to be hit so that the fielders can be positioned in the best locations. For this project, the team used applied machine learning techniques to predict the distribution of balls in play based on characteristics of the pitcher and batter. Their method substantially improved prediction accuracy – even in situations with limited historical data.
Nextracker (Abnormal Detection Methods Team)
Our Team: Tong Wang, Xinyue Wang
Faculty Mentor(s): Jeff Hamrick
Company Liaison(s): Chennan Li, Peng LiuProject Outcomes: The team developed abnormal detection methods for both solar and wind trackers and sensors. The team defined abnormal behaviors through time series models, including correlation coefficients and different notions of measuring “distance” in the data set.
Nextracker (Irradiance Forecasting Team)
Our Team: Lucas Oliveira
Faculty Mentor(s): Jeff Hamrick
Company Liaison(s): Chennan Li, Peng LiuProject Outcomes: The team developed a library for analyzing and optimizing the performance of control software for trackers. The team also developed libraries for preprocessing irradiance data and forecasting irradiance, using both statistical and deep learning models.
Nextracker (Solar Panel Design Team)
Our Team: Michael Reigelman
Faculty Mentor(s): Jeff Hamrick
Company Liaison(s): Chennan Li, Peng LiuProject Outcomes: This student performed exploratory data analysis to help engineers identify areas of improvement for new solar panel designs. The team created dashboards and libraries to enable engineers to continuously monitor specific features of the structural integrity of their designs.
Nisum
Our Team: Kyril Panilov
Faculty Mentor(s): Daniel O’Connor
Company Liaison(s): Ravi NarayananProject Outcomes: The team researched recommender systems and machine learning applications in finance. The team also implemented content-based filtering, collaborative filtering, and hybrid approaches to recommender systems. Finally, the team presented a recommender model to potential Nisum clients.
Oportun
Our Team: Wei He, Mengting Xu
Faculty Mentor(s): Jeff Hamrick
Company Liaison(s): Christine Walsh, Ajish GeorgeProject Outcomes: The team utilized multiple machine learning models to generate user engagement analytics and predict credit card transaction amounts. For another project, the team improved the customer identification matching system by building a set of rules and tracking evaluated metrics for the identification algorithm.
Orange
Our Team: Jih-Chin Chen, Derek Wolfgang Herwald
Faculty Mentor(s): David Guy Brizan
Company Liaison(s): Sarah LugerProject Outcomes: The team curated a dataset for a French-Bambara translation model by finding and cleaning existing translation data. This task involved researching aligners and implementing them into an alignment pipeline for unaligned data. It also included researching social strategies for annotation of untranslated Bambara data. The team then designed a Kaggle-style competition for the translation models. Finally, the team hyperparameter tuned byte pair encodings in light of a lack of available lemmatization.
Pocket Gems
Our Team: Shambhavi Gupta
Faculty Mentor(s): Daniel O’Connor
Company Liaison(s): Maxim Levet, Dixin Yan, Byron HanProject Outcomes: The team built and deployed language models to generate animation code scripts for content writers at Pocket Gems. The team also developed a churn prediction model to identify features contributing to player churn in a mobile game.
Propeller Health
Our Team: Cassidy Newberry, Anthony Wang
Faculty Mentor(s): Diane Woodbridge
Company Liaison(s): Ian Smeenk, Ben Theye, Connelly DoanProject Outcomes: The team developed a data pipeline to analyze screen usage for an application. The deployed dashboard was delivered to the internal product team for feature improvement and key performance indicator (KPI) evaluation.
Recology
Our Team: Dominnic Chant, Monashree Sanil
Faculty Mentor(s): Diane Woodbridge
Company Liaison(s): Minna Tao, Aijaz Patel, John LaBargeProject Outcomes: The team built a text classifier to automate the manual process of identifying customer locking accounts from comments data, using natural language processing (NLP) and machine learning models. Additionally, the team designed and developed a user interface to facilitate easy use of route sequencing tools. The team deployed their model as an application programming interface (API) on the Azure platform. Finally, the team designed and developed key performance indicators (KPIs) and Qlik Sense dashboards to help general managers optimize and manage routes more effectively.
Reddit
Our Team: Tongyao (Nancy) Ruan, Ka Yam
Faculty Mentor(s): Yannet Interian
Company Liaison(s): Mackenzie Greene, Jose Lobez, Deitrick Franklin, Cynthia LiProject Outcomes: Using A/B testing, the team analyzed how users interact with different interest groups across time, and assessed the depth of user interactions. The team developed a dashboard to share insights into the popularity of particular search terms and various topics among different interest groups.
Reputation
Our Team: Karsten Kao
Faculty Mentor(s): David Guy Brizan
Company Liaison(s): Kellie Meckenstock, Rui Li, Allie Akridge, Brad Null, Marine Lin, Sonika Cottmar, Hao XuProject Outcomes: The team achieved an improvement in neutral reviews’ recall by 87% (i.e., from 7.7% to 61.5%) by developing and tuning a Bidirectional Encoder Representations from Transformers (BERT) sentiment model. The team extended this project by building out an MLFlow pipeline for faster machine learning experimentation. Finally, the team built a Twitter text brand-extraction pipeline that improved recall by 19% after identifying issues in an analytics report by using Python.
Salk
Our Team: Fan Li, Chandrish Ambati
Faculty Mentor(s): Tahir Bachar Issa
Company Liaison(s): Uri ManoProject Outcomes: The team re-implemented a previously-published deep learning paper for super-resolution of brain microscope images using convolutional neural network (CNN) models built on FastAI and PyTorch. The team improved the quality of the resolution of the previous approach by using a perceptual loss function, combined with self-supervised learning techniques such as contrastive learning and inpainting.
Stanford Graduate School of Business
Our Team: Neset Aydin
Faculty Mentor(s): Steve Devlin
Company Liaison(s): Brian Chiver, Natalya Igorevna RapstineProject Outcomes: The team built an end-to-end automated extract/transform/load (ETL) pipeline using Python and the Redivis API to facilitate faculty data needs: for example, to scrape, organize, and store periodic Securities and Exchange Commission (SEC) reports available for faculty analysis in Redivis. The team also constructed tutorials and demonstrations to enable faculty to better use the pipeline functionality and Redivis platform.
Stanford Medicine
Our Team: Sneha Kumari, Sunil Kumar J S
Faculty Mentor(s): Michael Ruddy
Company Liaison(s): Sophia Ying Wang, Wendeng HuProject Outcomes: The team researched developing multimodal deep learning models to identify glaucoma patients who would need surgery in the near future. The team built a fusion model combining text data, image data, and structured data to enhance model performance. They also performed explainability studies to better understand which features the model relied upon to make predictions.
SubWiFi
Our Team: Arman Tavana, Kaihang Zhao
Faculty Mentor(s): Danielle Savage
Company Liaison(s): Michael TerryProject Outcomes: The team built a data pipeline to extract, transform and store user data using Python and Redis feature engineering, as well as feature extraction through BERT from users’ biographical data. The team deployed random forest, gradient boosting, and A/B testing to lift marketing campaign performance by approximately 15%.
Target
Our Team: Melvin Vellera, Chahak Sethi
Faculty Mentor(s): Diane Woodbridge
Company Liaison(s): Joey Jonghoon AhnnProject Outcomes: The team developed a recommendation system to create a bundle recommendation based on recipes using natural language processing (NLP) techniques. The output included ingredients, ingredient substitutes, and kitchen gadgets. Outputs were optimized based on quantity and personalized using the user’s dietary restrictions.
The Nature Conservancy
Our Team: Zhiyi Ren
Faculty Mentor(s): Michael Ruddy
Company Liaison(s): Kirk KlausmeyerProject Outcomes: The team predicted natural river flow estimates in the West Coast region to aid state agency staff in setting flow targets for efficient water management. The team used random forest models and techniques such as hyperparameter tuning and feature importance analysis to generate improved estimates of the monthly natural river flow data from the model. They also used natural language processing (NLP) algorithms to evaluate sustainability reports more efficiently.
University of California, San Francisco, Auto-Planning Radiosurgery
Our Team: Christopher Pang
Faculty Mentor(s): Yannet Interian
Company Liaison(s): Tomi NanoProject Outcomes: The team collaborated with researchers to build a deep learning model. This model takes three-dimensional brain tumors images (i.e., magnetic resonance images) and predicts the three-dimensional radiation shot locations using PyTorch and 3D U-Net.
University of California, San Francisco, Brain Metastasis
Our Team: Nestor Teodoro Chavez
Faculty Mentor(s): Yannet Interian
Company Liaison(s): Tomi NanoProject Outcomes: The team leveraged convolutional neural network (CNN) model architectures to accurately segment small lesions in the brain for radiosurgery. The project consisted of building upon an established auto-segmentation pipeline to increase the robustness of the model by using computer vision and deep learning techniques.
University of California, San Francisco, Chest X-Rays
Our Team: Charudatta Manwatkar
Faculty Mentor(s): Yannet Interian
Company Liaison(s): Tomi NanoProject Outcomes: The team developed a generative adversarial network (GAN) using PyTorch to enhance the visualization of cancer tumors in chest x-ray images. The team explored multiple deep learning architectures for paired (e.g., pix2pix) as well as unpaired (e.g., CycleGAN) image-to-image translation. Using a single-energy x-ray image as the model input, the model outputs a synthetic dual energy image with enhanced tumor visualization. The project should also help reduce patient exposure to dangerous x-rays.
University of California, San Francisco, Cognitive Decline
Our Team: Jeffery Ott, Chenjia Guo
Faculty Mentor(s): Yannet Interian
Company Liaison(s): Ashish RajProject Outcomes: Team team created a computer vision model to predict memory and speech degradation in dementia and Alzheimer’s patients. Using magnetic resonance imaging (MRI) scans from patients, the team created a pipeline to produce parcellation results, segmentation results, and cognitive scores in the hope of eventually speeding the diagnosis and treatment plans for patients suffering from cognitive decline.
University of California, San Francisco, Division of Gastroenterology
Our Team: Yangzhou Tang, Mitch Veele
Faculty Mentor(s): Shan Wang, Yannet Interian
Company Liaison(s): Vivek RudrapatnaProject Outcomes: The team collaborated with UCSF faculty to work on a pilot study of ulcerative colitis aiming to enhance inference from real-world data using an externally-derived missing data model. Students pre-processed clinical trial data in Python (pandas) and imputed missing data. Quality control and data harmonization were used to benchmark against original publications. Various classification algorithms were employed – logistic regression, random forest, XGBoost, etc. – to predict multiclass disease severity scores.
University of California, San Francisco, Division of Hospital Medicine
Our Team: Amanda Li Luo
Faculty Mentor(s): Shan Wang, Yannet Interian
Company Liaison(s): Xinran LiuProject Outcomes: The team collaborated with UCSF researchers to predict patient readmission rates. An extract/transform/load (ETL) pipeline was built using SQL, Python, and Spark for data exploratory analysis and model-building. Predictions on whether patients will be readmitted again within 30 days after discharge were performed by leveraging tools and techniques such as AutoML, logistic regression, random forest, gradient boosting, and XGBoost using the scikit-learn package.
University of California, San Francisco, Lung Cancer
Our Team: Lakshmi Manne, You Wu
Faculty Mentor(s): Yannet Interian
Company Liaison(s): Gilmer ValdesProject Outcomes: The team developed machine learning models for predicting toxicities of lung cancer patients treated with proton radiotherapy, taking advantage of the largest proton therapy database in the world. The team extracted features from medical image datasets and improved baseline models through feature engineering.
University of California, San Francisco, Natural Language Processing
Our Team: Haotian Gong, Ruifeng Luo
Faculty Mentor(s): Yannet Interian
Company Liaison(s): Jorge Ginart, Hui LinProject Outcomes: The team predicted the overall survival rate of brain tumor patients based on their electronic health record notes. The team built and calibrated neural network models – for example, Bidirectional Encoder Representations from Transformers (BERT) models, Long Short-Term Memory models, etc. To support their work, the team also refactored code, preprocessed data, and created data visualizations.
University of California, San Francisco, Oncology
Our Team: Young Zeng, Anish Mukherjee
Faculty Mentor(s): Michael Ruddy or Yannet Interian
Company Liaison(s): Benjamin ZiemerProject Outcomes: The team developed new cancer severity indices and predicted tumor growth in patients with brain metastases. The team used decision tree models to create interpretable severity indices and used random forest and gradient boosting models to predict survival. Additionally, the team utilized convolutional neural network (CNN) models to predict tumor growth using unstructured three-dimensional brain magnetic resonance imaging (MRI) data.
Velux
Our Team: Jeff Yeh
Faculty Mentor(s): Diane Woodbridge
Company Liaison(s): Jesper Frederiksen, Gabriele FustaProject Outcomes: The team implemented a data pipeline using the Kafka ecosystem to extract, process, and visualize data from Salesforce.
W.L. Gore
Our Team: Ashwani Rajan, Harshit Singh, Tanjin Sharma
Faculty Mentor(s): Daniel O’Connor
Company Liaison(s): Gen Gurczenski, Sharna Sattiraju, Vasudevan VenkateshwaranProject Outcomes: The team improved upon an internal PyTorch-based deep learning package to incorporate preprocessing pipelines and model architectures to support image segmentation tasks on microscopy and microCT data. The team used this package to build semantic segmentation workflows for histology and 3D-polymer images. Finally, the team refactored existing code to make use of PyTorch Lightning in order to increase usability, reproducibility and readability.
Walmart Labs
Our Team: Yanan Cao, Lawrence Lin
Faculty Mentor(s): Diane Woobridge
Company Liaison(s): Louise LaiProject Outcomes: The team implemented machine learning models to recommend grocery repurchases at Walmart’s e-commerce website. Additionally, the team developed a deep learning model for time-aware sequential recommendations.
-
ACLU Criminal Justice
Our Team: Qianyun Li
Goal: At the ACLU, the student identified potential discrimination in school suspensions by performing feature importance analysis with machine learning models and statistical tests.
ACLU Micromobility
Our Team: Max Shinnerl
Goal: At the ACLU, the student analyzed COVID-19 vaccine equitable distribution data. They developed interactive maps with Leaflet to visualize shortcomings of the distribution algorithm and automated the cleaning of legislative record data. They also developed a pipeline for storing data to enable remote SQL queries using Amazon RDS and S3 from AWS.
AWS
Our Team: Suren Gunturu
Goal: At AWS, the student employed machine learning techniques to interpret user natural language questions to SQL queries. They did this by interpreting features such as database information and input questions and mapped them to queries. They read available architecture on the topic and implemented them both from scratch using a Seq2Seq architecture as well as calling HuggingFace pretrained transformers for this task.
Bold
Our Team: Sophie Wang, Eriko Funasato
Goal: Students at Bold developed an end-to-end machine learning pipeline using Python’s Scikit-learn to classify churned customers. They also presented feature importance from the model to aid decision making. After being deployed in production, the pipeline increased the customer retention rate. Their work also included collaboration with the customer success team and performing A/B testing on email campaigns.
Boost
Our Team: Veeral Shah, Ricky Zhang
Goal: At Boost, students built and deployed a logistic regression pipeline to dynamically predict college basketball in-game win probability using Python and PostgreSQL. They established novel metrics for efficiency, excitement, and tension by analyzing mean, variance, and volatility trends of in-game win probability output.
Canal.ai
Our Team: Nicolas Decavel-Bueff, Taince Tan
Goal: Students at Canal.ai engineered and integrated machine learning techniques to perform NER as a tool to better collect and preprocess data. On another project, they worked on creating a content-based recommendation system to help identify competitors.
Cerenetics
Our Team: Zhimin Lyu, Victor Palacios, Daniel Carrera
Goal: At Cerenetics, students developed and deployed a Python multi-threading application for a brain functional MRI data preprocessing pipeline (DICOM- BIDS - normalized time series) to extract voxel signals and predict the presence of mental health disorders. They also created and implemented a novel Iterative Spectral Clustering algorithm for brain functional MRI voxel clustering.
Dictionary.com
Our Team: Emre Okcular, Yue Zhao
Goal: Students at Dictionary.com applied machine learning to website ad clicks and inner clicks data using Python's Scikit-learn and Matplotlib for visualization.
Electronic Arts
Our Team: Kexin Wang, Wenyao Zhang
Goal: At Electronic Arts, students built an anomaly detection process with supervised models (2D CNN) and improved model robustness with an unsupervised algorithm (Autoencoder) using Keras.
Eventbrite
Our Team: Yihong Shen, Jordan Uyeki
Goal: Students at Eventbrite used SQL and Python to compare revenue opportunities across different creator segments and to better understand creator behavior over time. They also compared various methods for event recommendation systems (collaborative filtering, networks, ERGM models, etc).
Facebook
Our Team: Zixi Luo
Goal: At Facebook, the student worked on the Facebook Community Product Group team to understand how businesses use Facebook groups. Their ultimate goal was to build a machine learning model to predict Facebook groups run by businesses and understand how they can improve the user experience.
Jumio
Our Team: Flora Chen, Hsuan-Yu Lin
Goal: At Jumio, students conducted EDA on identify thresholds that were effective at catching financial fraud. On another project, they built a flask app and set up modeling endpoints on AWS.
LaHaus
Our Team: Shiqi Tao, Rahul Bethavalli
Goal: Students at LaHaus employed NLP and deep learning techniques to identify description quality using Python. They also conceptualized and developed a suggestion system to recommend the most relevant custom page tags for real estate listings using a probabilistic random forest model. This resulted in an increase in the click-through rate by 70% post-deployment in production. On another project, they worked on improving the existing image captions for listings and leveraged zero-shot transfer learning of CLIP from OpenAI to generate qualitative and diverse captions. They implemented the end-to-end production pipeline using AWS, Pytorch, OpenAI, and Airflow.
LexisNexis
Our Team: Ye Tao, Michelle JanneyCoyle
Goal: At LexisNexis, students used machine learning techniques to perform legal analytics and conducted a deep learning model for a classification and text generation task. Additionally, they used matrix factorization to build a recommendation system in Python, and on another project they built a deep learning NLP API accessed by distributed spark job.
MedStar
Our Team: Catie Cronister
Goal: At MedStar, the student built a deep learning model to predict the proper radiology protocol that a physician would prescribe and authored a paper based on their work.
Metromile
Our Team: Weronica Green, Huidon Xu
Goal: Students at Metromile built and deployed a deep learning-based end-to-end computer vision system to identify vehicle quality issues using Resnet in PyTorch. They used the model predictions to run statistical analysis on various business metrics using SQL and Python. Lastly, they created an app that allows stakeholders to interact with the model predictions.
Metropolitan Transportation Commission
Our Team: Okeefe Niemann, Danh Nguyen
Goal: At the Metropolitan Transportation Commission, students created data pipelines to both organize and quality check jurisdiction entries. In addition, they created and fine-tuned deep learning models to classify buildings into zones.
New York Mets
Our Team: Moh Kaddoura, Trevor Santiago
Goal: Students at the New York Mets created an outfield defense model using multivariate distributions, powerful classifiers (RF and XGBoost) and clustering. They also used SciPy and NumPy to create a matchup model that accurately predicts success rates for a certain batter against a certain pitcher, or vice versa.
Novi Connect
Our Team: Vaishnavi Kashyap, Phillip Navo, Sandhya Kiran Reddy Donthireddy
Goal: At Novi, students engineered a pipeline to automate extraction of applicable columns from Excel files using Pandas and FuzzyMatch. Additionally, they conducted funnel analysis to understand customer engagement with the company platform. On another project, they leveraged Google Data Studio and Google Analytics and powered web analytics dashboards with high-level Business metrics and user engagement.
PG&E
Our Team: Tian Qi, Matthew Hui
Goal: Students at PG&E conducted exploratory data analysis to discover power outage patterns and employed machine learning techniques in order to identify assets that experience high risk events in the future using Python, SQL, AWS and Plantir Foundry.
Phylagen
Our Team: Audrey Barszcz
Goal: At Phylagen, the student utilized multiple machine learning models along with Shap feature importance to identify a subset of features that were the most predictive for classifying an outcome. On another project, they trained embeddings using a GloVe neural network model on genetic sequences.
Pocket Gems
Our Team: Yi Huang, Siwei Ma
Goal: Students at Pocket Gems used reinforcement learning to build a dragon agent that flies, follows and attacks in unity. They also developed a search engine and web server from scratch with NLP techniques.
Propeller Health
Our Team: Noah Matsuyoshi
Goal: At Propeller Health, the student predicted early life failures of sensors for medical device monitoring using Redshift (SQL) and Python.
Ranker
Our Team: Yueling Wu, Hashneet Kaur
Goal: At Ranker, students prototyped a video recommendation engine using LightFM’s collaborative filtering model based on users' implicit feedback on various website events such as trailer viewed or item clicked / added to watchlist. On another projects, they generated a script to minimize the "position on list" bias issue using descriptive statistics and SQL to increase reliability of crowd-sourced lists, performed audit on the current ranking algorithm, and identified discrepancies for the engineering team to resolve. They also identified trending shows by scraping data from Twitter, applying NLP techniques (e.g., parts of speech (POS) analysis, fuzzy string matching and sentiment analysis) and leveraging number of tweets and sentiment score.
Recology
Our Team: Amee Tan, Shruti Roy
Goal: Students at Recology automated sequencing of garbage pickup using telematics data, DBSCAN Clustering and Haversine Distance calculation in Python. On another project, they predicted garbage collection time using XGBoost and Isolation Forest.
Reddit
Our Team: Lucia Page-Harley, Maruo Napoli
Goal: At Reddit, students built a time series forecasting dashboard to understand and predict different video metrics. On another project, they performed analyses using SQL and Python visualizations to understand the German user-base at Reddit and planned/analyzed experiments to improve their product experience.
Stanford Graduate School of Business
Our Team: Kaiqi Guo
Goal: At the Stanford Graduate School of Business, the student explored different approaches such as BERT to detect and correct error in digitization of historical documents.
Stanford Medicine
Our Team: Daniel Blessing, Victor Nazlukhanyan
Goal: Students at the Stanford Medicine Department of Radiology conducted deep learning research and implemented computer vision methods to synthetically produce contrast-enhanced MRI images. Architectures included generative adversarial networks and U-Nets.
Syrup.tech
Our Team: Anni Liu, Aneri Dand
Goal: Students at Syrup.tech employed machine learning techniques to forecast sales for Syrup's retailer clients. They used Jinja3 and Plotly to build dashboards for tracking metrics, providing insights to retailers, as well as logging the results of machine learning experiments.
The Schmidt Family Foundation 11th Hour mBio Project
Our Team: Elyse Cheung-Sutton, Yingtong Lin, Eileen Wang, Remi LeBlanc
Goal: Students at the Schmidt Family Foundation's 11th Hour mBio project built web scrapers used on websites for African GMOs, IRS financial data, and news articles and created visualizations displaying the scraped information. They built a website to serve the analysis results using React and Django and trained a language model using fast.ai and Pytorch to support classification of African news articles. In order to serve information about the uses of agricultural biotechnology, they also consolidated data into one central hub to serve through a web application and deployed this containerized web application with Docker.
UCSF Brain Networks Laboratory
Our Team: Christabelle Pabalan
Goal: At UCSF, the student used computer vision and deep learning techniques, including multitask learning and ensemble learning, to predict cognitive scores for Alzheimer's patients.
UCSF Department of Radiation Oncology - Brain Metastasis
Our Team: Berkay Canogullari, Tianxiang Zhou
Goal: Students at UCSF predicted the outcome (local failure and patient survival) for large brain metastasis treated with radiation. The project consisted of performing tumor segmentation using deep learning followed by extraction of imaging features for prediction of treatment outcomes.
UCSF Department of Radiation Oncology - Prostate Cancer
Our Team: Jared Mlekush, Shuyan Li, Dashiell Brookhart, Min Che
Goal: Students at UCSF worked with physicians to predict the likelihood of success of salvage radiation treatment to help oncologists determine treatment options for prostate cancer patients. They utilized logistic regression, Cox Proportional-Hazards models, and feature importance analysis to create Kaplan-Meier estimators for patients. They also analyzed physician’s notes to create a predictive model for determining diagnostic error using techniques from Natural Language Processing (NLP) including Bag of Words and Word2vec and Machine learning models such as Random Forest, XGBoost, and Logistic Regression.
UCSF Department of Radiation Oncology - Spinal Metastatic Cancer
Our Team: Evan Chen
Goal: At UCSF, the student engaged in medical image preprocessing and deep learning (image segmentation) utilizing Python, SQL, Linear/Logistic Regression, more advanced Machine Learning, and Radiation Oncology treatment planning software.
UCSF Department of Radiation Oncology - Auto-Planning Radiosurgery
Our Team: Sicheng Zhou, Christopher Pang
Goal: At UCSF, students built a data pipeline to automatically generate datasets for cross-validation by pulling samples from main dataset. They developed deep learning solutions to generate high quality synthetic x-ray images from Digitally Reconstructed Radio-graphs (DRRs) images using Cycle-Consistent Generative Adversarial Networks (CycleGAN), which improves middle frequency power, an image quality score, by 20% on average compared with baseline Histogram Matching. This model could improve real-time x-ray imaging tracking during radiation therapy. They also visualized and compared synthetic x-ray images and Fourier Analysis results using customized HTML and Jinjia templates with Flask framework and presented the results to principle investigators.
UCSF Division of Hospital Medicine - Hospital Stays
Our Team: Patrick Poon, Boliang Liu
Goal: Students at UCSF collaborated with UCSF researchers to feature engineer and query patient's information using SQL and Spark. With the data, multiple machine learning models were used to forecast the need of the administration of antibiotics for these patients in 2-3 days using information from the first 24 hours utilizing Logistic Regression, Random Forest, XGBoost, and neural networks in PyTorch.
Virgo
Our Team: Efrem Ghebreab, Anawat Putwanphen
Goal: Students at Virgo developed a classification system for Ulcerative Colitis and Crohn's Disease utilizing deep learning and video image processing techniques.
W.L. Gore & Associates - Project 1
Our Team: Youchen Zhang, Kristofor Johnson
Goal: Students at W.L. Gore & Associates deployed Deep Learning Computer Vision techniques with Python's PyTorch package to segment microscopic images. They also built a Python package for internal deployment to easily train new models and architectures on different hyperparameters.
W.L. Gore & Associates - Project 2
Our Team: Grant Phillips, Stephen Embry
Goal: Students at W.L. Gore developed deep learning models to perform image classification, image segmentation, and keypoint detection on cornea image datasets using PyTorch.
W.L. Gore & Associates - Project 3
Our Team: Luke Thomas
Goal: At W.L. Gore, the student built a table extraction and merger system leveraging an AWS service for OCR, and IPython Widgets as a GUI.
Wanamaker
Our Team: Zachary Dougherty
Goal: At Wanamaker, the student developed architecture for analyzing and preprocessing Google Analytics data through a Markov chain attribution model.
Washington State University Basketball
Our Team: Kyle Brooks, Joshua Majano
Goal: Students at Washington State University utilized web scraping technologies to scrape international league data to be utilized in a model to predict an international player's projected performance in the NCAA. Additionally, they built out models to predict the same performance metric for NCAA transfer players.
-
ABC News
Our Team: Daren Ma, Ming-Chuan Tsai, Haree Srinivasan
Goal: Students at ABC News used Python to write a machine learning model to predict election results and used Docker and AWS to deploy the pipeline.
Accountability Counsel
Our Team: Jacob Goffin
Goal: At Accountability Counsel, Jacob created web-scraping scripts in Python & Selenium to build a first-of-its-kind database of human rights complaints. He also built a document-search (using Django/ElasticSearch) on thousands of .pdf documents, allowing users to quickly find relevant human rights cases to support their research.
Airbnb
Our Team: Ivette Sulca, Hoda Noorian
Goal: Students at Airbnb developed an evaluation tool prototype that identifies socioeconomic bias on Airbnb algorithms and experiments. They analyzed past A/B tests and built a dashboard using Python and Superset.
Beam
Our Team: Esther Liu, Jack Dong
Goal: At Beam Solutions, students used machine learning techniques to classify transaction data and perform text clustering. They also worked on industry research and database mapping for potential new customers.
Cuyana
Our Team: Hannah Lyon
Goal: At Cuyana, Hannah used Markov chains to develop a data-driven marketing attribution model that informed marketing spend. She created a customer propensity model using gradient boosting to determine critical site features that were then enhanced by the digital team to improve conversion. Additionally, she combined SQL and Tableau data for ad-hoc analysis of payment methods, trained neural networks to produce product embeddings used for a recommendation system on website product pages, and modeled repeat purchaser behavior predicting second purchases.
Eventbrite
Our Team: Maxine Liu, Zhentao Hou
Goal: Students at Eventbrite built a classifier and a deep learning model to improve event recommendations. They also researched cases for and against investing in online events from the perspectives of opportunity size, product data, and potential revenue impact. On another project, they analyzed text data with NLP libraries to identify features that are indicative of event listing quality.
Faire
Our Team: Kevin Wong
Goal: At Faire, Kevin developed a SQL-based outlier flagging mechanism. Additionally, he conducted a deep-dive analysis of the effectiveness of the Faire mobile app on retailer behavior using SQL, python, statistics, and propensity-score matching.
FLYR
Our Team: Peng Liu, Wenjie Duan
Goal: Students at FLYR developed a SQL/python workflow that predicted flight revenue by finding similar flights with clustering and Random Forest models.
FracTracker
Our Team: Vivian Chu
Goal: Vivian worked with FracTracker on the collection and aggregation of oil and gas data for the state of California, before conducting production analysis of oil wells at the pool level. Financial data was then added to predict the status of each of the oil wells as an asset or liability.
Golden State Warriors
Our Team: Kyrill Rekun, Xueying Li
Goal: At the Golden State Warriors, students used machine learning techniques to create a last-minute ticket buyer model that predicts the probability of a person being a last-minute, planner, or in-between buyer. Using the lifetimes Python package, they built a proxy lifetime value spend model for customers to aid in marketing and ticket targeting. These projects utilized tools such as Pandas, Seaborn, and sklearn.
Gore Medical
Our Team: Peng Liu, Wenjie Duan
Goal: Students at Gore Medical developed PyTorch CNN models using the fast.ai API to detect key points in medical optical coherence tomography images, thus allowing for automated assessment of an implant. They achieved these results using transfer learning and data augmentation.
Hohonu
Our Team: Ariana Moncada, Matthew Sarmiento
Goal: At Hohonu at the University of Hawaii, students created a tidal forecasting pipeline that helps populate a Django web application and Plotly plots for forecasts. They clustered multiple time series datasets together to increase the performance of their multivariate time series models in R and Python.
Human Rights Data Analysis Group (HRDAG)
Our Team: Bing Wang
Goal: At the Human Rights Data Analysis Group (HRDAG), Bing gleaned critical location of death information from unstructured text fields in Arabic using Google Translate and Python Pandas, adding identifiable records to Syrian conflict data. She wrote R scripts and bash Makefiles to create blocks of similar records on killings in the Sri Lankan conflict to reduce the size of search space in the semi-supervised machine learning record linkage (database de-duplication) process.
Manifold
Our Team: Shreejaya Bharathan, Geoffrey Hung
Goal: Students at Manifold developed a Python library that utilizes machine learning and deep learning to solve for the parameters of dynamical systems defined by differential equations using PyTorch, Docker and MLFlow.
Metromile
Our Team: Matthew King, Lin Meng
Goal: At Metromile, students created a crash classification model to predict the primary point of impact during a collision using telematics data collected from customers. On another project, they used deep learning to classify images of fraudulent cars.
New York Mets
Our Team: Rushil Sheth
Goal: At the New York Mets, Rushil created infield and outfield shift models using multivariate distributions, powerful classifiers (RF and XGBoost) and clustering.
Metropolitan Transportation Commission (MTC)
Our Team: Kamron Afshar, Michael Schulze
Goal: Students at MTC used deep learning to train a Neural Net Image Classifier on images of buildings to classify their use. They generated the data set using Google API. They also built a Selenium crawler data pipeline that scrapes legal codes and collected them in a Redshift database to track changes.
NakedPoppy
Our Team: Lisa Chua, Shane Buchanan
Goal: At NakedPoppy, students improved the recommendation system for new customers by incorporating content-based and collaborative filtering trained on clickstream data. They used NLP techniques to extract key aspects from Google reviews and implemented feature-based opinion mining on product reviews to assist in the scoring of new products. Later, they conducted market basket analysis on transaction data to provide customers with “pair with” recommendations and increase engagement.
Baltimore Orioles
Our Team: Collin Prather
Goal: At the Baltimore Orioles, Collin implemented a Deep Recurrent Survival Analysis model (LSTM in PyTorch) to predict the probability that an American League manager will remove their pitcher using in-game time series data. Another prominent project was developing a model to predict relief pitchers’ level of fatigue, then deploying a containerized (Docker) web application on AWS to host the model and explanatory visualizations to communicate the analysis to key stakeholders in the Orioles front office.
PG&E
Our Team: Kathy Yi, Sean Sturtevant, Jingwen Yu, Nithish Kumar Bolleddula
Goal: Students at PG&E used SQL, Python and AWS Sagemaker to employ machine learning techniques to predict whether or not a PG&E asset is likely to experience a failure. On another project at PG&E, students built computer vision models on drone imagery to identify defects in power grid lines.
Phylagen
Our Team: Nicholas Parker, Mundy Reimer
Goal: Students at Phylagen worked on projects with data from microbiome samples and laboratory processes that involved software development, data analysis, and machine learning.
Pocket Gems
Our Team: Qingmengting Wang, Tian (Arthur) Qin
Goal: At Pocket Gems, students completed two NLP projects using LSTM and Dialogflow.
Propellor Health
Our Team: Andrew Eaton, Xuxu Pan
Goal: Students at Propellor Health built a Random Forest model to predict how long it would take to solve a customer support ticket using word embeddings from the ticket texts and a Continuous Bag of Words (CBOW) model. They also published live dashboards with information on ticket counts and complaint rates on a Tableau Server.
Recology
Our Team: Yunzheng Zhao, Shishir Kumar
Goal: At Recology, students used linear regression to generate route statistics and service time estimation from GIS and trash collection data. They also analyzed routing data and identified anomalies in the reporting and data-capturing process.
Reddit
Our Team: Kevin Loftis, Esme Luo
Goal: Students at Reddit worked on graph-based subreddit community detection. They developed a subreddit graph based on user view overlap and performed community detection on graph to cluster similar subreddits using Python and NetworkX. This doubled the subscription rate of subreddits compared to the existing system. On another project, they worked on a streaming feature extraction pipeline where they architected and developed a Flink streaming data processor in Scala using Docker, Flink, Kafka, Circle CI, and Kubernetes.
Reputation
Our Team: Meng Lin, Hao Xu
Goal: At Reputation, students used entity matching in deep learning for matching addresses and performed topic modeling to analyze topic trends in reviews.
Salk Institute for Biological Sciences
Our Team: Alaa Abdel Latif, Annette (Zijun) Lin
Goal: Students at the Salk Institute for Biological Studies built super-resolution deep learning models using fast.ai and PyTorch.
Sparta Science
Our Team: Sunny Kwong
Goal: At Sparta Science, Sunny worked on improving the reliability of balance tests by performing multiscale entropy analysis with R and Python on force plate scans.
Specialty's Cafe & Bakery
Our Team: Jiaqi Chen, Sakshi Singla
Goal: At Specialty's Cafe & Bakery, Jiaqi performed revenue forecasting employing time series analysis and EDA and also worked on building a recommendation engine using machine learning.
Stanford Graduate School of Business
Our Team: Jingxian Li
Goal: Students at the Stanford Graduate School of Business cleaned SEC 10-K documents and built word2vec models based on this corpus. They also came up with different ways to evaluate models and learned to use the BERT model.
Trulia
Our Team: Lea Genuit, Alan Flint
Goal: At Trulia, Lea employed deep learning techniques using Pytorch to identify rotated scanned documents by a factor of 90 degrees. She also implemented an improvement of the current solution (Tesseract, an OCR engine) by working on a patch of the image using Python. Then, she compared the results of Tesseract and the CNN models. On another project at Trulia, Alan built a power analysis tool in Python for Trulia's A/B testing platform. This entailed coding and deploying an ETL pipeline and designing an interactive application using Streamlit. His second project involved employing an interpretable machine learning model to identify site features that influence positive outcomes for interested home buyers.
TruStar
Our Team: Dillon Quan
Goal: At TruStar, Dillon built parsers to normalize data ingested into the data lake to centralize samples into one format for predictive analytics usage downstream using Spark and Scala. His second project focused on analyzing URLs and how to generate scores to determine their level of maliciousness using Python and Pytorch.
UCSF Brain Networks Laboratory
Our Team: Qingyi Sun, Akanksha
Goal: Working with the Brain Networks Laboratory at UCSF and the Wicklow AI in Medicine Research Initiative (WAMRI), students focused on characterizing diseases, such as Autism and Alzheimer’s disease, making diagnosis and prognosis from multi-channel brain Magnetoencephalography (MEG) data. They built an LSTM (Long Short-Term Memory) model using PyTorch to analyze brain MEG data and extract information to make predictions on characteristic parameters of interest. On another project, they worked on pretraining 3D Convolutional Neural Networks with brain MRI data. The models were pretrained using a segmentation task.
UCSF Bakar Computational Health Sciences Institute
Our Team: Linqi Sheng
Goal: Working with UCSF and the Wicklow AI in Medicine Research Initiative (WAMRI), Linqi built an LSTM (Long Short-Term Memory) model using PyTorch to analyze brain MEG data, extract information, and make predictions on characteristic parameters of interest.
UCSF Radiation Oncology Department the Wicklow AI in Medicine Research Initiative (WAMRI)
Our Team: Roja Immanni
Goal: Working with the UCSF Radiation Oncology Department, Roja found that medical image datasets are fundamentally different from natural image datasets in terms of the number of available training observations and the number of classes for the classification task. She hypothesized that compared to architectures used for natural images, those needed for medical imaging can be simpler. She proposed smaller architectures and showed how they perform similarly while significantly saving training time and memory. This is joint work with Gilmer Valdes at UCSF.
UCSF and the Wicklow AI in Medicine Research Initiative (WAMRI)
Our Team: Zachary Barnes
Goal: Working with UCSF and the Wicklow AI in Medicine Research Initiative (WAMRI), Zachary used UCSF's Spark environment for EHR data to create a data set, generate labels for hospital acquired sepsis patients, and create prediction models using sklearn and Pytorch.
UCSF Morin Lab and the Wicklow AI in Medicine Research Initiative (WAMRI)
Our Team: Sihan Chen
Goal: Working with the Morin Lab at UCSF and the Wicklow AI in Medicine Research Initiative (WAMRI), Sihan built a 3D Residual U-net to precisely segment metastases from brain MRI images with PyTorch. He evaluated the effects of number, size, and locations of metastases on the accuracy, which has resulted in a scientific conference presentation and a manuscript and helped UCSF design a state-of-the-art model.
Vasant Lab at UCSF and the Wicklow AI in Medicine Research Initiative (WAMRI)
Our Team: Shrikar Thodla
Goal: Working with the Vasant Lab at UCSF and the Wicklow AI in Medicine Research Initiative (WAMRI), Shrikar worked on multiple projects. These included using deep learning to segment and classify medical images, attempting to generate 3D images from multiple 2D image views, leading migration of full-stack components from GCP to IBM, detecting accidental rotations in images using CNNs built in PyTorch, and optimizing code to read images from a database.
United Health Care
Our Team: Srikar Murali, Sean Tey
Goal: Students at United Healthcare cleaned and processed millions of insurance claims transactions with SQL and did hypothesis testing on demographics-related data. On another project, they predicted members who are likely to be hospitalized in the near future as part of a system for identifying administratively complex members with a Gradient Boosting Trees model using the CatBoost library.
Valimail
Our Team: Andrew Young, Charles Siu
Goal: At Valimail, students tackled the problem of classifying a backlog of 100K+ unknown internet domains generated by Valimail Defend. They developed an end-to-end machine learning pipeline that classifies trusted domains by detecting whether they belong to low-risk categories such as real estate. The Gradient Boosting Machine (GBM) model achieved a 95%+ precision rate with test data when classifying real estate domains using Natural Language Processing (NLP) for web content analysis. On another project, they designed and implemented REST APIs using Flask in Dockerized modules in the pipeline and built web scrapers using BeautifulSoup to gather multiple external data sources for ML model training.
Virgo
Our Team: Mikio Tada, Stephanie Jung
Goal: Students at Virgo developed a Python script to extract data frames from 120 hours of video. They used Google AutoML to train deep learning models to automate video recording during endoscopic medical procedures and to develop an automatic procedure type tagging system. On another project, they built a prototype object detection tool for real-time polyp tracking during a colonoscopy using CVAT for data labeling and Google AugoML to train the deep learning model.
Walmart Labs
Our Team: Samarth Inani, Akansha Shrivastava
Goal: At Walmart Labs, students developed an image inpainting tool to remove occlusions from high-resolution furniture images using partial convolutions. They also worked on a research-oriented project to enhance the color detection algorithm to improve the accuracy of the color attribute in the product description of furniture listed on Walmart.com using Pytorch and Open-CV.
Wicklow AI in Medicine Research Initiative (WAMRI) and Medstar Georgetown University Hospital
Our Team: Max Calehuff, Xintao (Todd) Zhang, Wendeng Hu
Goal: Students working with the Wicklow AI in Medicine Research Initiative (WAMRI) and MedStar Georgetown University Hospital used NLP to create an automated grading program for medical student imaging reports.
Zyper
Our Team: Andy Cheon, Aakanksha Nallabothula Surya
Goal: At Zyper, students built and deployed an image classification convolutional neural network (CNN) with PyTorch to help brands efficiently recruit fans with desired aesthetic types on social media. They applied feature importance methods using machine learning in Python to identify top factors that drive engagement rates of user-generated content. They also developed a user location prediction pipeline using NLP tools (NLTK, spaCy) to improve upon the existing location predictor and discovered and visualized trends from group chat content from 15 brand communities using mainly Pandas and ggplot.
-
Aleinvault
Our Team: Sankeerti Haniyur
Goal: On this project, the student employed deep learning & NLP techniques to automatically tag cybersecurity documents. She then built a named entity recognition model to detect indicators of compromise in the documents.
Beam Solutions
Our Team: Darren Thomas, Liying Li
Goal: Students employed NLP techniques in Python for name recognition and used Pytorch and an LSTM to detect fraudulent transactions. On another project, scraped data using restful API, creating an application using Flask in Python. They also applied unsupervised machine learning models to build clustering and anomaly detection models using Python.
General Electric
Our Team: Benjamin Khuong, Ziqi Pan
Goal: Students worked on an object detection project to detect defects in CT scans of machine parts. Their project was focused on designing computer vision based solutions for automatic defect-detection on industrial devices. They implemented state of the art deep learning algorithms such as Faster R-CNNs, R-FCNs, and 3D convolutional neural networks.
Bolt Threads
Our Team: Wenkun Xiao, Nicole Kacirek
Goal: Students worked closely with the marketing team to optimize campaign messages by applying NLP and machine learning techniques to competitors’ product reviews and social media posts. They also built and productionised a CLTV (customer lifetime value) and revenue prediction model which was put into production.
Check Point/Dome9
Our Team: Brian Chivers, Evan Liu
Goal: Students developed an unsupervised learning algorithm to detect anomalies in AWS network traffic.
Dictionary.com
Our Team: Rebecca Reilly, Minchen Wang
Goal: Students focused on increasing revenue using topic modeling, employing Python and the spaCy library to discover industry relationships using advertiser behavior. They employed machine learning technologies to predict online ad prices and identify important features. On another project, they created an NLP classifier to correctly identify acceptable and appropriate sentences.
Eventbrite
Our Team: Nan Lin, Lance Fernando
Goal: Students built machine learning models to predict the LTV (lifetime value) of customers. On another project, they deduplicated over 5 million venue addresses using fuzzy string similarity metrics and a HMM, then utilized this data to create a search ranking method to recommend venues to event creators.
Fair
Our Team: Aditi Sharma, Zhi Li
Goal: Students built a content-based recommendation system for cars and employed auction price prediction.
Fandom
Our Team: Byron Han, Yuhan Wang
Goal: Students used SQL to extract data from AWS, then employed NLP techniques to build a text classification pipeline.
Hohonu
Our Team: Connor Swanson
Goal: The student built anomaly detection systems in Python for environmental data. He also built time series forecasting models to predict future environmental shifts and built dashboards to host their findings.
Kiva
Our Team: Tyler Ursuy, Anush Kocharyan
Goal: Students classified each Kiva partner into risk categories by implementing a Random Forest risk detection model that monitors the financial, geographic, and economic information of Kiva’s global partners. They also built an interactive online dashboard to provide easy access to data analyses, data visualizations, and model predictions which will help Kiva reduce the amount of time and money spent on manually inspecting partner information and conducting scheduled in-person visits.
KWH Aanalytics
Our Team: Hongdou Li, Zhe Yuan
Goal: Students employed machine learning techniques to predict solar panel performance across the country and provided business inference.
Leanplum
Our Team: Hai Le, Jon-Ross Presta
Goal: Students automated the data generation process for a dashboard with a Python script. They also trained an NLP model which takes the subject line, information about the app that sends the email, and information about the recipient segment to predict email open rates using PyTorch. On another project, the students used Python/PyTorch to build an NLP model to predict user engagement based on message content.
Manifold AI
Our Team: Edward Richard Owens, Prakhar Agrawal
Goal: Students created a system that optimizes the operation of HVAC systems by detecting the stabilization of building temperature from sensor data. On another project, they built a golf simulator with the model utilizing a video of a person hitting a golf ball and outputting the ball’s trajectory using machine learning and physics. They employed methods and architectures such as background removal, darknet (YOLO) and optical flow for computer vision.
Mantaray
Our Team: Shivee Singh, Xiao Han
Goal: Students used machine learning and deep learning to identify microplastics in the ocean water using OpenCV Python and PyTorch. Their main focus was to build object detection models trying to locate microfibers from underwater images to approximate the total volume and distribution of microfibers in the ocean.
Metromile
Our Team: Christopher Olley, Wei Wei
Goal: Students used machine learning and deep learning to identify drivers based on their telematics data (speed and acceleration). On another project, the students extracted events and created features based on this data to train tree based models using Python. They extracted labeled trip data from SQL and Amazon S3 storage and built the ML/DL models to identify users using Python and SQL.
Mozilla
Our Team: Sarah Melancon, Brian Wright
Goal: Students used Python and Spark to combine and aggregate add-on related data from a variety of data sources into a single data source. They also built a dashboard based on this data source using Redash. The students built an ETL pipeline that aggregated several data sources into one combined dataset.
Metropolitab Transportation Commission
Our Team: Jacques Sham, Quinn Keck
Goal: Students built a data lake on AWS, involving S3 and Redshift, using tools available in the market (Trifacta and Python). On another project, they analyzed Clipper and FasTrak data, tracked key performance indicators, and built dashboards. They developed machine learning and times series models to predict daily Clipper Card usage within 4%.
Delta Analytics
Our Team: Chong Geng
Goal: The student developed metrics to define the success of the product in terms of user engagement and answering efficiency. He also applied NLP techniques to upgrade the recommender system and built a dashboard to visualize the results.
Naked Poppy
Our Team: Nina Hua, Donya Fozoonmayeh
Goal: Students employed machine learning for product recommendations and used PySpark to apply a model in a distributed environment. They also implemented machine learning techniques to classify skin color from an image and worked a recommendation system to improve user experience.
Orange Silicon Valley
Our Team: Evan Calkins, Jinghui Zhao, Ran Huang
Goal: Students developed an algorithm to support targeted marketing campaigns, which identifies similar mobile users based on their location patterns. They built an n-gram language model for the African language of Wolof to improve functionality of a chatbot using Python. On another project, they calculated relative store location optimality by comparing user movements and travel patterns using a large dataset (4TB) of mobile user information processed on a 9-node Spark cluster.
Pacific Electric and Gas Company
Our Team: Gokul Krishna Guruswamy, Louise Lai
Goal: Students used PyTorch to train deep learning object detection and classification models to identify faults in equipment and to detect small-scale objects in millions of large drone images. They worked extensively in AWS cloud environment (EC2, S3, lambda, SageMaker, etc.) to productionize these models.
Recology
Our Team: Paul Kim, Katja Wittfoth
Goal: Students used deep learning techniques to identify different types contaminants in waste bins. They also automated identification of contaminants in complex images of waste bins by developing a multi-label image classification model using deep learning, Pytorch, Python, and AWS.
Recology (Routes)
Our Team: Xu Lian, Philip Trinh
Goal: Students built a machine learning model to predict a truck's accident occurrence using Sklearn. They used data analytics and machine learning methods to provide policy recommendations on how Recology can increase safety when collection drivers are out in the city. They also merged sheets from different sources using Pandas and PySpark.
Reddit
Our Team: Yixin Sun, Julia Amaya Tavares
Goal: Students built a machine learning pipeline on Airflow to estimate subreddit retention ability. They used Python spaCy package to build a small tool to extract keywords from post comments. On another project, they used TensorFlow to create a multi-label classifier for post titles, and SQL / Pandas for data acquisition and pre-processing.
Reputation.com
Our Team: Randy Ma, Xi Yang
Goal: Students developed a review sentiment classifier using a deep learning model with LSTM and Self-Attention to improve reputation assessment (Python, PyTorch). They extracted customer concerns by building a multi-gram keyword extraction tool using syntactic dependency analysis. They also built an automated operational insight reporting tool (SQL, Python) to assess strengths & weaknesses of the client’s user experiences.
San Francisco County Transportation Authority
Our Team: Crystal Sun, Marwa Oussaifi
Goal: Students created web-based visualization tools for presenting the number of accessible jobs and trip patterns within San Francisco with D3.js. They automated complex data preprocessing and data pipelines to accommodate different scenarios when collecting, processing and piping the data using python. On another project, they implemented different ML algorithms to predict auto ownership per household.
Split.io
Our Team: Xinran Zhang, Zitong Zeng
Goal: Students developed a Scala notebook to help the customer service team analyze user-retention metrics such as DAU and Return Retention. They provided an anonymization routine for sensitive impressions and events data using Spark UDF and Murmurhash3. They explored alternatives to traditional parametric tests to improve the performance credibility of A/B test analysis. They also researched and implemented outlier detection methods in Scala.
Trulia
Our Team: Xinke Sun, Jyoti Prakash Maheswari
Goal: Students used SQL to track KPIs and built tables to store daily metrics using Python. The students applied deep learning techniques to understand the content of real-estate listings consisting of images and text and to predict lead submission.
Trustar Technology
Our Team: Viviana M. Peña-Márquez, Neha Tevathia
Goal: Students built an NLP model to identify the malware names using CBOW model and leveraged the open source data from Twitter. They used Pytorch to build the CBOW model. Created and implemented pipeline to automatically collect tweets using Twitter’s API, applied machine learning and natural language processing algorithms to detect entities, and feed daily detections to a dashboard.
Ubisoft
Our Team: Tian Qi, Jessica Wang
Goal: The students deployed a machine learning pipeline to predict the paid users within the next two weeks using Python and SQL. In another project, the students predicted short term purchase using Python.
UCSF Department of Neurology (Neuroscape Lab)
Our Team: Jenny Kong
Goal: The student used machine learning with fMRI data to classify network patterns of concurrently activating brain regions that arise during successful high-fidelity memory retrieval.
UCSF Department of Radiation Oncology (AI)
Our Team: Miguel Romero Calvo
Goal: The student employed deep learning techniques to improve the performance of Neural Networks in small data. He also conducted research on training and transfer learning methodologies.
UCSF Department of Radiation Oncology (Computer Vision Lab)
Our Team: Anish Dalal, Robert Sandor
Goal: Students employed deep learning techniques in computer vision to accurately segment ventricles in the brain using Pytorch. On another project, they built a text classifier that predicts cancer patient survival from physician notes using Python, PyTorch, Bash, and FastAI.
UCSF Department of Radiation Oncology (Quantitative Imaging Lab)
Our Team: Alan Perry, Tianqi Wang
Goal: Using Python, students employed deep learning techniques to make segmentation of different organs, to make dose volume diagnosis, and to achieve MRI to CT images transformation.
UCSF Division of Cardiology (Arnaout Laboratory)
Our Team: Max Alfaro, Divya Bhargavi
Goal: Students built deep learning models to classify different views of echocardiograms. They performed exploratory data analysis to become familiar with medical terminology.
Ultimate Software
Our Team: Victoria Suarez, Harrison Mamin
Goal: Students built recommender system to predict which matched candidates to job posting using Python, which improved recruiters' efficiency by 56%. They researched methods of detecting unconscious gender bias in performance reviews using word embeddings and neural networks. On another project, the students worked on two approaches to extract causal language pairs from text; one using a deterministic rule-based engine and one using a neural network, integrating them into a web-based UI using Flask.
Under Armour
Our Team: Adam Reevesman, Meng-Ting Chang
Goal: Students built a rule-based algorithm to identify when a user finished a route but forgot to stop their tracker in the MapMyFitness app using Python. They also preformed functions related to EDA.
United Health Care
Our Team: Tomohiko Ishihara, Maria Vasilenko
Goal: Students gathered user reviews on Personal Health Record apps on Apple App Store and Google Play Store and used Latent Dirichlet Analysis to try to see what app features users talk about most. They built models to predict whether a member is likely to get pregnant by creating a data set, performing feature engineering and building machine learning models. On another project, they collected user reviews from GooglePlay and Appstore and performed topic modeling (LDA) as implemented in Gensim.
Valimail
Our Team: Joy Qi, Jialiang Shi
Goal: Students built machine learning classification models to identify lists of legitimate email domains versus fraudulent email domains. They employed machine learning techniques to classify whether an unknown domain is trusted or untrusted. On another project, they created scraping script to scrape social links on web pages.
Valor Water Analytics
Our Team: Yihan Wang, Jian Wang
Goal: Students predicted water utility customer nonpayment with a Random Forest model and implemented the model in Python into Valor’s codebase. They segmented utility customers with K-means clustering to understand their behavior. On another project they applied multiple time series model for identifying malfunctioned water meters. They used SQL and Python to build end-to-end workflow for the project.
Vida Health
Our Team: Shulun Chen
Goal: The student used SQL, Python, and Swagger to build data pipelines.
Wiser Solutions
Our Team: Ziyu Fan
Goal: The student applied data science and machine learning techniques to forecast E-commerce retailer sales using Python. On another project, she used machine learning and NLP to find anomalies in product matching.
Zume Pizza
Our Team: Brian Dorsey, Fiorella Tenorio
Goal: Students used Python, TensorFlow, and Time Series demand prediction models. They worked on a model to predict the probability of client purchases and a demand prediction model.
-
Capital One
Our Team: Arpita Jena, Devesh Maheshwari, Alexander Howard
Goal: Students employed NLP and deep learning techniques to classify sensitive information in Capital One's internal domain using Python.The result was wrapped in a Flask web app. Another project involved software engineering with the goal of automating Capital One's AWS authentication process.
Cogitativo, Inc
Our Team: Yiqiang Zhao, Gongting Peng
Goal: Students employed machine learning methods to build a data pipeline for anomaly detection. They also used Python for data exploration.
Delta Analytics
Our Team: Stephen Hsu
Goal: Students worked within a multidisciplinary team to offer data science services to a nonprofit organization. Specifically, students developed an NLP-based model in Python to classify forum posts so that forum questions could be appropriately matched with professionals who are best positioned to answer them.
Endgame
Our Team: Timothy Lee
Goal: Students did data pipeline work using the Python API service. Their work involved classification of PDF files using Python XGBoost and the collecting of research data samples using Python.
Eventbrite
Our Team: Holly Capell Students at Eventbrite used machine learning in Python to model ticket sell-through rates in order to help the company identify platform features that drive event sell-out. They performed cohort analyses using Python to help understand the revenue life-cycle of Eventbrite customers and investigated seasonality in ticket sales, using SQL to query data and R to create data visualizations.
Firest Republic Bank
Our Team: Bingyi Li, Christopher Csiszar
Goal: Students built a web-based system to classify municipal bonds in order to assure government compliance using Python and Flask. They used big data analytics, machine learning and clustering algorithms to automate the classification of the bank's municipal bond portfolio into High Quality Liquid Asset bonds. This work replaced the need for inefficient and costly external consultants to perform this task quarterly.
FLYR
Our Team: Yue Lan, Akshay Tiwari
Goal: Students wrote SQL scripts to perform exploratory data analysis and built a data pipeline to ingest airline customer data. They also employed machine learning techniques to build and validate models using python to predict bookings and cancellations of airline tickets as part of the Flyr airline revenue management system They also worked on another project that used machine learning techniques to predict customer budget and price sensitivity.
Houston Astros
Our Team: Jake Toffler
Goal: Students clustered individual pitchers' pitches by pitch type using level-set trees, a density-based clustering method, in Python.
Isazi Consulting
Our Team: Shikhar Gupta, Fei Liu
Goal: Students used deep learning CNN techniques to identify diseases in chest X-rays.
Kiva
Our Team: Ting Ting Liu, Jose Antonio Rodilla Xerri
Goal: Students employed machine learning techniques to identify relevant factors that may affect whether or not a Kiva loan will reach full funding. They developed a web application powered by a random forest model in order to predict the success of loans, highlight which factors are driving those loans, and provide suggestions on how to improve them.
Manifold
Our Team: Vinay Patlolla, Jason Carpenter
Goal: Students worked on two projects with Manifold. In the first project, they used machine learning models such as Logistic Regression, Random Forest and XGBoost to detect faults in oil pipeline using Python. In the second project, they developed a multi-camera multitracking pipeline to track people in a scene using deep learning and clustering techniques.
Metromile
Our Team: Chenxi Ge
Goal: Students worked on a complex computer vision problem using deep learning with the goal of locating characters to decode the character sequence.
Mozilla
Our Team: Tyler White, Jing Song
Goal: Students used Spark to obtain data to build a public-facing Firefox Health report dashboard. They used time series analysis to predict ESR usage and checked the validity of t-tests with non-parametric tests.
MTC
Our Team: Danai Avgerinou, Shannon McNish
Goal: Students worked on a data engineering project to build a small centralized data warehouse to host MTC's data. They also worked on a data science project using NLP with FastTrak survey data and made discoveries involving ridership patterns of Clipper users.
Nextdoor
Our Team: Natalie Ha, Christopher Dong
Goal: Students built a text classification model to categorize survey responses and found correlations with NPS. On another project, they built a Tableau dashboard for funnel analysis on reported content in the platform. They also built and deployed (with Airflow) a machine learning model using Spark ML to predict survey text responses and created complex SQL queries to calculate metrics regarding content moderation.
Orange
Our Team: Guoqiang Liang
Goal: Students employed machine learning techniques to assign probabilities of churn using Python and Spark. On another project, they used NLP techniques to classify legal documents.
Our Team: Ernest Kim, Davi Alexander Schumacher
Pocket Gems
Our Team: Dixin Yan, Spencer Stanley
Goal: At Pocket Gems, students employed machine learning techniques to build a churn model and a matchmaking model for a newly developed game. They also researched and developed models to help the marketing team with channel attribution and creatives optimization. On another project, they used time series methods to predict the impact of paid advertising channels on organic install volume.
Price F(X)
Our Team: Neerja Doshi, Alvira Swalin
Goal: Students employed machine learning (Python) and deep learning (PyTorch) techniques to build a product recommendation system.
Recology
Our Team: Khoury Ibrahim, Danielle Savage
Goal: Students used deep learning techniques to build a multi-label image recognition CNN using PyTorch to identify contaminants in images of landfill, recycling, and compost in Recology's images of waste.
Reputation.com
Our Team: Sara Mahar, Nicha Ruchirawat
Goal: Students automated the real-time detection of a data feed failure from Google, Bing and Facebook sources using a suite of standardized hypothesis tests. On another project, they identified significant clusters of words from tens of thousands of omni-channel reviews with Latent Dirichlet Allocation (LDA) topic modeling and k-means clustering.
San Francisco 49ers
Our Team: Kishan Panchal
Goal: Students used machine learning techniques to create a weekly cohort-based churn prediction system for season ticket holders. On another project, they created a data ingestion system to get external ticket data into the team's data warehouse.
San Francisco County Transportation Authority
Our Team: John Rumpel, Kaya Tollas
Goal: Students used Python to compute accessibility metrics for transit stops (this was later used in their study on TNCs and ridership). On another project they prepared data for input into the SFCTA travel model. And on another project they visualized traffic incidents with an interactive map using javascript.
SEGA
Our Team: Mathew Shaw, Cara Qin
Goal: Students employed machine learning techniques to identify suspicious users, predict LTV, and classify game themes.
SF17
Our Team: Daniel Grzenda, Jade Yun
Goal: Students employed graph theory to quantify variants and analyze protein data from the blood of patients using Python.
Snaplogic
Our Team: Nimesh Sinha, Zizhen Song
Goal: Students used natural language processing and machine learning techniques to build a data pipeline recommendation engine. On another project, they worked on clustering customers based on login data.
Stanford Graduate School of Buisness
Our Team: Ker-Yu Ong, Chen Wang
Goal: Students compared cloud databases (AWS, Google Bigquery, Snowflake and Databricks) by running benchmarking queries for research use cases. They also ran machine learning models to classify WSJ articles and used NLP techniques to extract information from news articles and identify topics in Amazon product reviews.
Swiftly
Our Team: David Kes
Goal: Students developed an exponentially weighted moving average (EWMA) control charting scheme to detect bus detours for a variety of transit agencies using Python. The algorithm was used to help automate the customer success team's process for detecting defaults in any transit agencies systems.
Tally
Our Team: Thy Khue Ly, Beiming Liu
Goal: Students used machine learning to predict default risks of customers and also to cluster them into groups based on their credit card transactions using Python. On another project they used NLP to predict transaction categories, and on a final project they used time-series and machine learning to predict user annual income with transactional data.
Ubisoft
Our Team: Feiran Ji, Lingzhi Du
Goal: Students predicted users’ purchasing behavior for future games using machine learning techniques and deployed an end-to-end pipeline to put the model into production on Hadoop clusters using Spark. Additionally, they visualized insights and developed an interactive dashboard to be used in conjunction with the predictive model.
UCSF
Our Team: Siavash Mortezavi, Kerem Can Turgutlu
Goal: Students used traditional machine learning techniques to predict overall survival of meningioma cancer patients and used deep learning and computer vision to automatically segment brain structures.
UCSF
Our Team: Sangyu Shen, Qian Li
Goal: Students employed machine learning techniques to classify patients with side effects from radiation therapy using Python.
Under Armour
Our Team: Ryan Campa, Zhengjie Xu
Goal: Students used machine learning to predict stride and cadence to help runners improve their form. They also used unsupervised learning to identify organized race events from millions of rows of workout data.
United Health Care
Our Team: Savannah Logan, Sooraj Mangalath Subrahmannian
Goal: Students applied NLP techniques in Python to identify the main complaints in a website survey. They then employed machine learning techniques to identify areas of possible improvement in coverage rejection time.
Valimail
Our Team: Taylor Pellerin, Devin Bowers
Goal: Students employed machine learning techniques to help identify fraudulent email sending behavior. They prototyped internal tooling, documentation, and more. Additionally, they built a machine learning classifier to help identify new legitimate email services. This allows Valimail to quickly scan through email aggregate reports to identify legitimate services that email on a customer's behalf.
Valor Water Analytics
Our Team: Jingjue Wang, Kunal Kotian
Goal: Students trained a recurrent neural network to forecast water consumption and flagged unusual water meter readings by comparing the deviation of forecasts from true values. They wrote production code for a pipeline to extract and transform data, train deep learning models using TensorFlow, and generate forecasts for several water consumption time series.
Vida Health
Our Team: Nishan Madawanarachchi, Chengcheng Xu
Goal: Students predicted weight loss among customers using linear regression with R. On another project, they used logistic regression in Python to predict the urgency level of clients' messages using logistic regression in Python. They also built a chat bot which aimed to help new users with the onboarding process.
Voodoo Sports
Our Team: Ford Higgins, Ian Pieter Smeenk
Goal: Students contributed to a 'football genome' project for stylistic classification of teams using Python. They built a college basketball statistical model that builds on top of existing models in order to improve them and designed tools for football coaches to use to as an aid in scouting opposing teams. These projects were completed using Python, R, SQL and D3.js.
Vungle
Our Team: Deena Liz John, Patrick Yang
Goal: Students used Python, SQL and Looker to implement A:B testing at Vungle, revolving around the comparison of different ad templates, levels of compression, and more. They also aided in the development of an in-house A:B testing platform.
Wiser Solution
Our Team: Liz Chen, Yu Tian
Goal: Students developed an end-to-end pipeline in Python using computer vision and deep learning technologies for a company promotional product to recognize online promotions from images. On another project, they deployed REST APIs into production and designed experiments to compare the results from different methods.
Xoom
Our Team: Vanessa Zheng
Goal: Students developed fraud detection models on a high-dimensional imbalanced dataset using Python. On another project, they devised and evaluated global risk metrics to monitor, condition and strengthen fraud models with SQL & Python.
Zipcar
Our Team: Sri Santhosh Hari
Goal: Students used time series techniques to forecast customer churn. Additionally, they used machine learning techniques like Random Forest and XGBoost to identify key features affecting bookings to predict members' likelihood of booking a car.