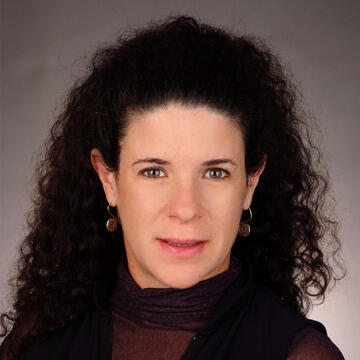
Yannet Interian
Associate Professor
Biography
Yannet is an associate professor in the Master’s in Data Science program, and her research interests lie in the application of machine learning and deep learning to medical data. She holds a PhD in applied mathematics from Cornell University and a BS in mathematics from the University of Havana, Cuba. After a postdoctoral fellowship at UC Berkeley, she worked for five years as a data scientist at Google. Yannet co-founded Akualab, a start-up that helped organizations develop data-driven products using machine intelligence and has designed data science courses for both UC Berkeley and USF.
Research Areas
- Machine learning
- Deep learning
- Machine learning applied to medicine
Education
- Cornell University, PhD in Applied Mathematics, 2006
Selected Publications
-
Cluceru, J., Phillips, J., Molinaro, A., Interian, Y., Luks, T., Alcaide-Leon, P., Nair, D., LaFontaine, M., Shai, A., Chunduru, P. and Pedoia, V., 2021. NIMG-25. IMPROVING THE NONINVASIVE CLASSIFICATION OF GLIOMA GENETIC SUBTYPE WITH DEEP LEARNING AND DIFFUSION-WEIGHTED IMAGING. Neuro-oncology, 23 (Supplement_6), pp.vi133-vi134.
-
Nano, T., Zhou, S., Interian, Y., Valdes, G., Morin, O. and Descovich, M., 2021, October. Generation of Synthetic X-ray Images Using Deep Learning for Evaluation of Real-time Tracking. In AMERICAN JOURNAL OF CLINICAL ONCOLOGY-CANCER CLINICAL TRIALS (Vol. 44, No. 10, pp. S141-S141). TWO COMMERCE SQ, 2001 MARKET ST, PHILADELPHIA, PA 19103 USA: LIPPINCOTT WILLIAMS & WILKINS.
-
Ma, L., Nano, T., Pang, C., Interian, Y., Valdes, G. and Morin, O., 2021, October. GammaKnife Auto-planning Using Artificial Intelligence: A Preliminary Comparison of ML and DL Treatment Planning Parameters for Vestibular Schwannoma. In AMERICAN JOURNAL OF CLINICAL ONCOLOGY-CANCER CLINICAL TRIALS (Vol. 44, No. 10, pp. S141-S141). TWO COMMERCE SQ, 2001 MARKET ST, PHILADELPHIA, PA 19103 USA: LIPPINCOTT WILLIAMS & WILKINS.
-
Valdes, G., Interian, Y., Morin, O. and Arbelo, W., 2021. PO-1791 Scaling Down Deep Learning Architectures for Medical Datasets. Radiotherapy and Oncology, 161, pp.S1516-S1517.
-
Cherifa, M., Interian, Y., Blet, A., Resche-Rigon, M. and Pirracchio, R., 2021. The Physiological Deep Learner: First application of multitask deep learning to predict hypotension in critically ill patients. Artificial Intelligence in Medicine, 118, p.102118.
-
Ginart, J.B., Ziemer, B.P., Nano, T., Turgutlu, K.C., Ibrahim, A., Interian, Y., Dalal, A., Sandor, R., Leseur, J., Vallières, M. and Upadhaya, T., 2021. Multi-Modal Brain and Ventricle Segmentation Using Weakly Supervised Transfer Learning.
-
Romero, M., Interian, Y., Solberg, T. and Valdes, G., 2020. Targeted transfer learning to improve performance in small medical physics datasets. Medical Physics, 47(12), pp.6246-6256.
-
Valdes, G., Romero, M., Interian, Y. and Solberg, T., 2020. PO-1527: When small is too small? Training Deep Learning models in limited datasets. Radiotherapy and Oncology, 152, p.S825.
-
Cluceru, J., Alcaide-Leon, P., Interian, Y., Pedoia, V., Phillips, J., Nair, D., Luks, T., Villanueva-Meyer, J. and Lupo, J., 2020. NIMG-36. AUTOMATIC STRATIFICATION OF ENHANCING AND NON-ENHANCING GLIOMAS INTO GENETIC SUBTYPES USING DEEP NEURAL NETWORKS AND DIFFUSION-WEIGHTED IMAGING. Neuro-Oncology, 22 (Supplement_2), pp.ii155-ii155.
-
Immanni, R., Valdes, G. and Interian, Y., 2020, June. Designing a Simple CNN Model in Terms of Size and Computational Complexity to Perform Classification Task On Medical Images. In MEDICAL PHYSICS (Vol. 47, No. 6, pp. E600-E600). 111 RIVER ST, HOBOKEN 07030-5774, NJ USA: WILEY.
-
Gennatas, E.D., Friedman, J.H., Ungar, L.H., Pirracchio, R., Eaton, E., Reichmann, L.G., Interian, Y., Luna, J.M., Simone, C.B., Auerbach, A. and Delgado, E., 2020. Expert-augmented machine learning. Proceedings of the National Academy of Sciences, 117 (9), pp.4571-4577.
-
Nano, T., Descovich, M., Hirata, E.Y., Interian, Y. and Valdes, G., 2020. Reducing IMRT QA Workload by 95% and Keeping the Same Level of Quality Control. International Journal of Radiation Oncology, Biology, Physics, 108(3), pp.e311-e312.
-
Romero, M., Interian, Y., Solberg, T. and Valdes, G., 2019. Training deep learning models with small datasets.
-
Ibrahim, K., Savage, D.A., Schnirel, A., Intrevado, P. and Interian, Y., 2019. ContamiNet: Detecting contamination in municipal solid waste. arXiv preprint arXiv:1911.04583.
-
Valdes, G., Romero-Calvo, M., Solberg, T. and Interian, Y., 2019, June. Deep Learning in Medical Physics: Reality Or Noise?. In MEDICAL PHYSICS (Vol. 46, No. 6, pp. E276-E276). 111 RIVER ST, HOBOKEN 07030-5774, NJ USA: WILEY.
-
Morin, O., Chen, W.C., Nassiri, F., Susko, M., Magill, S.T., Vasudevan, H.N., Wu, A., Vallières, M., Gennatas, E.D., Valdes, G., Pekmezci, M., Alcaide-Leon, P., Choudhury, A.,Interian, Y., Mortezavi, S., Turgutlu, K., Bush, N., Solberg, T., Braunstein, S., Sneed, P., Perry, A., Zadeh, G., McDermott, M., Villanueva-Meyer, J. and Raleigh, D., 2019. Integrated models incorporating radiologic and radiomic features predict meningioma grade, local failure, and overall survival. Neuro-oncology advances, 1(1), p.vdz011.
-
Interian, Y., Rideout, V., Kearney, V.P., Gennatas, E., Morin, O., Cheung, J., Solberg, T. and Valdes, G., 2018. Deep nets vs expert designed features in medical physics: an IMRT QA case study. Medical physics, 45(6), pp.2672-2680.
-
Interian, Y., Valdes, G., Vincent, R., Joey, C., Vasant, K., Olivier, M., Gennatas, E. and Solberg, T., 2018. EP-2163: Deep Neural Networks vs Medical Physicists: An IMRT QA case study. Radiotherapy and Oncology, 127, p.S1194.
-
Valdes, G., Chang, A.J., Interian, Y., Owen, K., Jensen, S.T., Ungar, L.H., Cunnan, A., Solberg, T.D., Hsu, I. (2018). HDR salvage brachytherapy: Multiple hypothesis testing vs. machine learning analysis. International Journal of Radiation Oncology Biology Physics.
-
Valdes, G., Interian, Y. (2018). Comment on 'Deep convolutional neural network with transfer learning for rectum toxicity prediction in cervical cancer radiotherapy: a feasibility study'. Physics in Medicine & Biology, 63(6).
-
Interian, Y., Rideout, V., Keanery, V.P., Efstathios, G., Morin, O., Cheung, J., Solberg, T. and Valdes, G. (2018). Deep Nets vs Expert-Designed Features in Medical Physics: An IMRT QA case study. Forthcoming from Medical Physics.
-
Sundar Dorai-Raj, Yannet Interian, Igor Naverniouk and Dan Zigmond. Adapting Online Advertising Techniques to Television. Online Multimedia Advertising: Techniques and Technologies, Information Science Reference, Hershey PA, 2011, pp. 148- 165 (Book Chapter).
-
Adrian Ulges, Yannet Interian, Luciano Sbaiz. Predictive Modeling of User Behavior on YouTube. (2011)
-
Catching a viral video Tom Broxton, Yannet Interian, Jon Vaver Vaver, Miriam Wattenhofer Catching a viral video. Journal of Intelligent Information Systems volume 40, issue 2, year 2013, pp. 241 - 259 (Shorter version IEEE SIASP@ICDM 2010).
-
H De Arazoza, R Lounes, T Hoang, Y Interian. Modeling HIV epidemic under contact tracing – the Cuban case. Computational and Mathematical Methods in Medicine 2 (4), 267-274, 2010.
-
Sundar Dorai-Raj, Yannet Interian, Dan Zigmond. Evaluating TV Ad Campaigns Using Set-Top Box Data. Re:Think 2010.
-
Yannet Interian, Sundar Dorai-Raj, Igor Naverniouk, P. J. Opalinski, Kaustuv, Dan Zigmond. Ad Quality On TV: Predicting Television Audience Retention. Proceedings of International workshop on Data Mining and Audience Intelligence for Advertising (ADKDD), 2009.
-
Dan Zigmond, Sundar Dorai-Raj, Yannet Interian, Igor Naverniouk. Measuring Advertising Quality on Television: Deriving Meaningful Metrics from Audience Retention Data. Journal of Advertising Research, vol. 49, 2009, pp. 419-428.
-
Yannet Interian, Kaustuv, Igor Naverniouk, P. J. Opalinski, Sundar Dorai-Raj, Dan Zigmond. Do Viewers Care? Understanding the impact of ad creatives on TV viewing behavior . Re:Think 2009.
-
Dan Zigmond, Yannet Interian, Steve Lanning, John Hawkins, Raimundo Mirisola, Simone Rowe, Yaroslav Volovich. When Viewers Control the Schedule: Measuring the Impact of Digital Video Recording on TV Viewership. Key Issues Forums at ARF Audience Measurement Conference, 2009.
-
Richard Durrett , Yannet Interian. Genomic Midpoints: Computation and Evolutionary Implications. (2006)
-
Yacine Boufkhad, Olivier Dubois, Yannet Interian, Bart Selman. Regular Random k-SAT: Properties of Balanced Formulas. J. Autom. Reasoning 35(1-3): 181-200, 2005.
-
Yannet Interian. Approximation Algorithm for Random MAX-kSAT. In International Conference on Theory and Applications of Satisfiability Testing SAT 2004. Full version in Springer-Verlag Lecture Notes in Computer Science, 173-182, 2004.
-
Hubie Chen, Yannet Interian. A Model for Generating Random Quantified Boolean Formulas. In Proceedings of the Nineteenth International Joint Conference on Artificial Intelligence (IJCAI), 66-71, 2005.
-
Yannet Interian. Backdoor Sets for random 3-SAT. In International Conference on Theory and Applications of Satisfiability Testing SAT 2003.